The Impact of Artificial Intelligence on Clinical Trial Recruitment
Clinical trials are the cornerstone of medical research, providing the empirical foundation for developing new treatments and therapies. They are essential for assessing the safety and efficacy of medical interventions, ranging from pharmaceuticals to surgical procedures and beyond. The outcomes of clinical trials directly influence regulatory approvals and clinical practices, making them critical for advancing healthcare. However, the process of conducting clinical trials is fraught with challenges, particularly in recruiting and retaining participants. Recruitment is a crucial phase that often determines the overall success and timeline of a trial. It involves identifying and enrolling eligible patients who meet specific criteria, which can be a complex and time-consuming task. Furthermore, ensuring that these participants remain engaged and complete the trial is equally challenging, often requiring significant resources and effort.
Overview of Artificial Intelligence (AI)
Artificial Intelligence (AI) refers to the simulation of human intelligence in machines programmed to think and learn. AI encompasses various technologies, including machine learning, natural language processing (NLP), and predictive analytics, which enable computers to process and analyze vast amounts of data more efficiently than humans. In recent years, AI has made significant inroads into various sectors, including healthcare. Its ability to handle complex datasets, identify patterns, and make predictions has positioned it as a transformative tool in medicine, from diagnostics to personalized treatment plans. In the context of clinical trials, AI is increasingly being used to optimize patient recruitment and retention, addressing some of the longstanding challenges in these areas.
The Challenges in Clinical Trial Recruitment and Retention
Recruitment Challenges
Recruiting participants for clinical trials is often a bottleneck that can delay study timelines and increase costs. One of the primary challenges is identifying eligible participants. This process requires sifting through vast amounts of medical records, screening patients for specific criteria, and ensuring they meet the trial's inclusion and exclusion criteria. The manual nature of this process can lead to inefficiencies and errors, further complicating recruitment efforts. Additionally, achieving diverse demographic representation is another significant challenge. Clinical trials need to include participants from various age groups, genders, ethnic backgrounds, and geographic locations to ensure the results are generalizable. However, certain populations are often underrepresented in clinical trials, leading to potential biases in the data and limiting the applicability of the study findings. Moreover, regulatory and ethical considerations add another layer of complexity. Clinical trials must comply with strict regulations to protect participants' rights and safety, which can sometimes conflict with recruitment goals.
Retention Challenges
Retention of participants in clinical trials is as crucial as recruitment. Ensuring that participants stay engaged and complete the trial is vital for the study's validity and reliability. One of the main challenges in retention is maintaining participants' interest and commitment throughout the trial, which can last for months or even years. Dropout rates can significantly affect the study's outcomes, leading to incomplete data and potentially skewed results. Addressing participants' concerns, such as inconvenience, side effects, or lack of perceived benefit, is essential to mitigate dropout risks. Additionally, providing adequate support and communication throughout the trial can help keep participants motivated and informed.
How AI is Transforming Patient Recruitment
AI in Identifying Eligible Participants
AI has the potential to revolutionize the way eligible participants are identified for clinical trials. By leveraging data mining and natural language processing (NLP) technologies, AI can analyze vast amounts of medical data, including electronic health records (EHRs), medical literature, and patient registries. This capability allows AI to quickly and accurately identify patients who meet the specific criteria for a clinical trial. For instance, NLP can be used to extract relevant information from unstructured data, such as doctors' notes or pathology reports, to identify patients with specific medical conditions or treatment histories. Predictive analytics, another AI technology, can also play a crucial role in identifying potential candidates for clinical trials. By analyzing patterns and trends in historical data, predictive models can forecast which patients are more likely to meet the trial criteria or benefit from the intervention. This proactive approach can significantly reduce the time and resources required for patient screening, accelerating the recruitment process.
Enhancing Diversity and Inclusion
Diversity and inclusion are critical components of clinical trials, ensuring that the results are applicable to a broad population. AI can help enhance diversity in clinical trials by using algorithms for demographic analysis. These algorithms can analyze data from various sources, including census data and social media, to identify underrepresented populations and target recruitment efforts accordingly. For example, AI can help identify geographic areas with a high prevalence of a particular condition or demographic group, allowing researchers to focus their recruitment efforts in those regions. Additionally, AI can assist in designing more inclusive trials by identifying potential barriers to participation, such as language or cultural differences, and suggesting strategies to overcome them. For instance, AI-powered chatbots can provide information and answer questions in multiple languages, making it easier for non-English-speaking participants to learn about and join a trial.
Streamlining Recruitment Processes
AI is also streamlining the recruitment process by automating various tasks, such as patient matching and outreach. Traditional recruitment methods often rely on manual processes, such as screening medical records or conducting phone interviews, which can be time-consuming and labor-intensive. In contrast, AI can automate these processes, significantly reducing the time and costs associated with recruitment. For example, AI algorithms can automatically match patients to clinical trials based on their medical history, demographic information, and other relevant factors. Additionally, AI-powered platforms can automate outreach efforts, such as sending personalized invitations to potential participants or providing information about the trial. This automation not only speeds up the recruitment process but also ensures a more consistent and targeted approach, improving the likelihood of enrolling eligible participants.
Improving Patient Retention with AI
Personalized Patient Engagement
Retaining participants in a clinical trial is crucial for its success, and AI can play a significant role in enhancing patient engagement. AI-driven communication tools, such as chatbots and virtual assistants, can provide personalized and timely interactions with participants. These tools can answer questions, provide reminders, and offer support throughout the trial, helping to keep participants informed and engaged. For example, AI chatbots can send personalized reminders for upcoming appointments or medication schedules, reducing the likelihood of missed visits or non-compliance. Additionally, virtual assistants can provide educational content tailored to each participant's needs and preferences, helping them understand the importance of their participation and stay motivated.
Monitoring and Predictive Analytics
AI can also enhance patient retention by monitoring participants' adherence to the trial protocol and their health status. By analyzing data from wearable devices, EHRs, and other sources, AI can provide real-time insights into participants' behavior and well-being. This monitoring can help identify potential issues, such as non-adherence to the trial protocol or emerging health concerns, allowing researchers to intervene proactively. Predictive analytics can also play a crucial role in preventing dropouts by identifying participants at risk of discontinuing the trial. For instance, AI models can analyze historical data to identify patterns associated with dropout risk, such as specific demographic factors or adverse events. By identifying these risk factors early, researchers can take targeted actions to address participants' concerns and improve retention rates.
Enhancing Patient Experience
Enhancing the patient experience is another key area where AI can make a significant impact. Virtual trial platforms, which use AI technologies, offer a more flexible and convenient alternative to traditional, in-person trials. These platforms enable remote monitoring and data collection, allowing participants to complete assessments or report symptoms from the comfort of their homes. This flexibility can be particularly beneficial for participants with mobility issues or those living in remote areas, making it easier for them to participate in the trial. Additionally, AI-powered feedback systems can provide continuous support and guidance, helping participants navigate the trial process and address any concerns or questions they may have. This ongoing support can improve participants' overall experience and satisfaction, increasing the likelihood of their continued involvement in the trial.
Case Studies and Real-World Applications
Successful AI-Driven Recruitment Campaigns
Several real-world examples demonstrate the successful use of AI in clinical trial recruitment. For instance, a major pharmaceutical company used AI-driven data analytics to identify potential participants for a study on a new diabetes treatment. By analyzing EHRs and other medical data, the AI system identified patients with specific characteristics, such as a particular range of blood sugar levels and a history of using certain medications. This targeted approach not only expedited the recruitment process but also ensured that the study included a diverse group of participants. Another example is a research institution that used AI-powered chatbots to engage potential participants for a mental health study. The chatbots provided information about the study, answered questions, and guided participants through the enrollment process. This approach significantly increased the number of participants recruited, particularly among younger demographics who are more comfortable with digital communication.
AI Tools and Platforms in Use
Several AI tools and platforms are currently being used to support clinical trial recruitment and retention. For example, IBM Watson for Clinical Trial Matching is an AI-powered platform that uses NLP and machine learning to match patients to clinical trials based on their medical records. The platform can analyze large volumes of data and identify patients who meet the specific criteria for a trial, streamlining the recruitment process. Another example is Deep 6 AI, a platform that uses AI to search and analyze EHRs, clinical notes, and other medical data to identify eligible participants. The platform can also identify potential issues, such as missing data or inconsistencies, and suggest solutions to address them. Additionally, several companies are developing AI-powered chatbots and virtual assistants to support patient engagement and retention. These tools can provide personalized communication, reminders, and support, helping to improve participants' experience and adherence to the trial protocol.
Lessons Learned and Best Practices
The use of AI in clinical trial recruitment and retention has provided several valuable lessons and best practices. One key lesson is the importance of data quality and accuracy. AI systems rely on large volumes of data to make predictions and identify patterns, so ensuring the accuracy and completeness of this data is crucial. Another lesson is the need for transparency and explainability in AI decision-making. Participants and researchers need to understand how AI algorithms make decisions, particularly when it comes to identifying eligible participants or predicting dropout risks. Providing clear explanations and justifications for these decisions can help build trust and confidence in the AI system. Additionally, it's important to consider ethical and regulatory considerations when using AI in clinical trials. Ensuring data privacy and security, avoiding bias in AI algorithms, and maintaining transparency are all critical to the ethical use of AI in this context.
Ethical and Regulatory Considerations
Data Privacy and Security
The use of AI in clinical trials raises several ethical and regulatory considerations, particularly concerning data privacy and security. AI systems often require access to large volumes of sensitive data, including medical records and personal information, to make accurate predictions and recommendations. Ensuring the privacy and security of this data is crucial to protect participants' rights and prevent unauthorized access or breaches. Compliance with data protection regulations, such as the General Data Protection Regulation (GDPR) in Europe and the Health Insurance Portability and Accountability Act (HIPAA) in the United States, is essential. These regulations set strict guidelines for data collection, storage, and use, requiring organizations to implement robust security measures and obtain informed consent from participants.
Ethical Use of AI in Recruitment
The ethical use of AI in clinical trial recruitment involves ensuring fairness, transparency, and accountability. One key concern is avoiding bias in AI algorithms, which can lead to discriminatory practices or exclude certain populations from the recruitment process. For example, if an AI algorithm is trained on biased data, it may be more likely to select participants from specific demographic groups, leading to a lack of diversity in the trial. To address this issue, it's essential to use diverse and representative datasets for training AI models and to regularly audit and evaluate the algorithms for potential biases. Additionally, transparency in AI decision-making processes is crucial. Participants and researchers need to understand how AI systems make decisions, particularly when it comes to identifying eligible participants or predicting dropout risks. Providing clear explanations and justifications for these decisions can help build trust and confidence in the AI system.
Future Trends and Innovations
Advancements in AI Technologies
The field of AI is continuously evolving, with new technologies and techniques emerging regularly. One promising area is the use of deep learning, a type of machine learning that involves neural networks with multiple layers. Deep learning has shown great potential in various applications, including image and speech recognition, and is now being explored in clinical trials. For example, deep learning algorithms can analyze complex medical images, such as MRI scans or pathology slides, to identify potential participants for trials. Another emerging technology is reinforcement learning, a type of machine learning that involves training algorithms to make decisions based on feedback from their actions. Reinforcement learning has the potential to optimize various aspects of clinical trials, from patient recruitment to treatment protocols.
Integration of AI with Other Technologies
AI is also being integrated with other emerging technologies to enhance clinical trial processes. For example, the Internet of Things (IoT) involves connecting various devices and sensors to collect and transmit data in real time. In clinical trials, IoT devices can monitor participants' vital signs, medication adherence, and other health-related data, providing valuable insights for researchers. Blockchain technology is another area of interest, particularly for ensuring data security and transparency. Blockchain can create a secure and immutable record of all transactions and data exchanges, making it ideal for maintaining the integrity of clinical trial data. By integrating AI with IoT and blockchain, researchers can create more efficient, secure, and transparent clinical trial processes.
The Future of Patient-Centric Trials
The future of clinical trials is likely to be more patient-centric, focusing on the needs and preferences of participants. AI will play a crucial role in this transformation by providing personalized and tailored experiences for participants. For example, AI can help design personalized treatment plans based on participants' genetic information, medical history, and other factors. Additionally, virtual trial platforms, powered by AI, will offer more flexibility and convenience for participants, allowing them to participate in trials from anywhere. This shift towards patient-centric trials will not only improve the experience for participants but also enhance the quality and relevance of the data collected.
Notable Labs: Transforming Clinical Trial Recruitment with AI
Notable Labs is at the forefront of leveraging Artificial Intelligence (AI) to revolutionize clinical trial recruitment and retention. Clinical trials are essential for advancing medical research, providing crucial data on the safety and efficacy of new treatments. However, recruiting and retaining participants can be challenging, often delaying studies and increasing costs. This is where AI steps in, offering innovative solutions to streamline these processes.
AI technologies, such as machine learning and natural language processing (NLP), enable Notable Labs to sift through vast amounts of medical data swiftly and accurately. This capability helps in identifying eligible participants more efficiently than traditional methods. Moreover, AI-driven predictive analytics allow for a proactive approach, forecasting potential candidates who are most likely to meet the trial criteria. This reduces the time and resources needed for recruitment, ensuring studies commence promptly.
Furthermore, Notable Labs utilizes AI to enhance diversity and inclusion in clinical trials. By analyzing demographic data, AI algorithms identify underrepresented groups and target them effectively, ensuring a broad representation of participants. This not only improves the generalizability of the study findings but also addresses ethical considerations around equity in research.
Retention of participants is another critical aspect of clinical trials that Notable Labs addresses using AI. AI-driven communication tools, such as chatbots and virtual assistants, provide personalized engagement, reminders, and support, keeping participants informed and motivated. Additionally, AI monitors participants' adherence to the study protocol and their overall health status, allowing for timely interventions to prevent dropouts.
In conclusion, Notable Labs is transforming the landscape of clinical trial recruitment and retention with its AI-powered solutions. By improving efficiency, enhancing diversity, and personalizing participant engagement, Notable Labs is paving the way for more effective and inclusive clinical research, ultimately accelerating the development of new therapies and treatments.
Conclusion
Artificial Intelligence (AI) is revolutionizing clinical trial recruitment and retention, addressing many of the longstanding challenges in these areas. AI technologies, such as data mining, natural language processing (NLP), and predictive analytics, are transforming the way eligible participants are identified, recruited, and retained in clinical trials. These technologies are enhancing the efficiency and effectiveness of recruitment processes, improving diversity and inclusion, and providing personalized support and engagement for participants.
The potential of AI to revolutionize clinical trials is immense. By leveraging AI, researchers can streamline various aspects of the trial process, from recruitment and retention to data analysis and monitoring. AI can also provide valuable insights and predictions, helping researchers make more informed decisions and optimize trial outcomes. Moreover, the integration of AI with other emerging technologies, such as IoT and blockchain, promises to create more secure, transparent, and efficient clinical trial processes.
As the field of AI continues to evolve, it is crucial for stakeholders in the clinical trial industry to embrace these technologies and explore their potential benefits. By investing in AI-powered tools and platforms, organizations can enhance their recruitment and retention efforts, improve the quality of their data, and ultimately accelerate the development of new treatments and therapies. However, it is also essential to address the ethical and regulatory challenges associated with the use of AI in clinical trials, ensuring that these technologies are used responsibly and transparently. As we move towards a more patient-centric approach to clinical trials, the role of AI will undoubtedly become even more critical, helping to shape the future of medical research and healthcare.
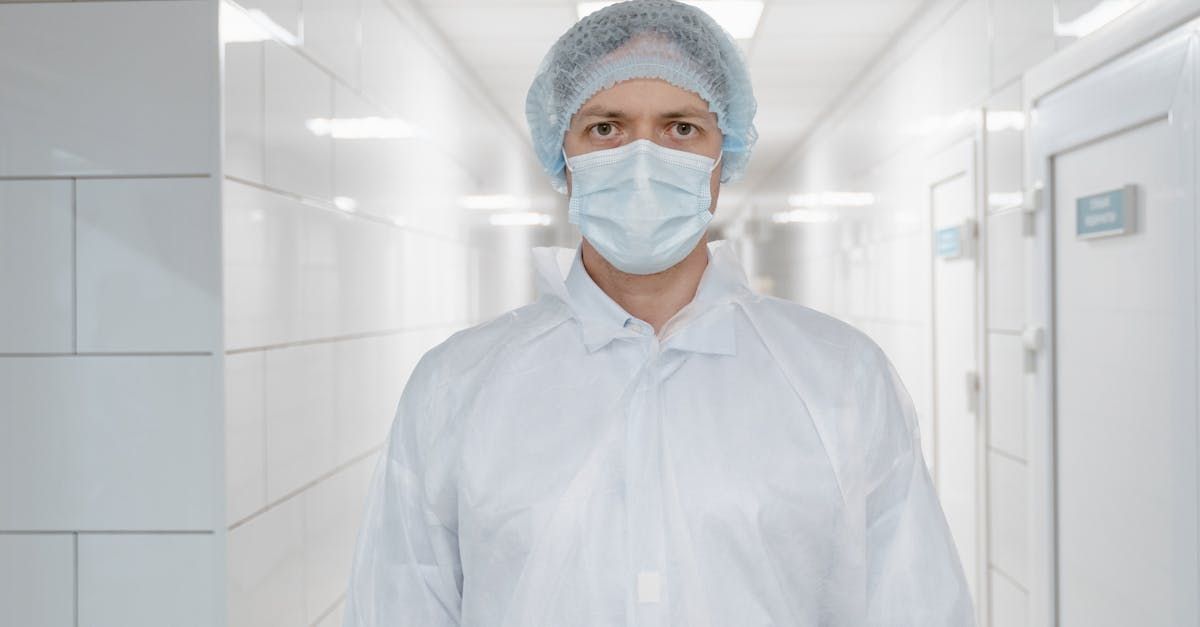
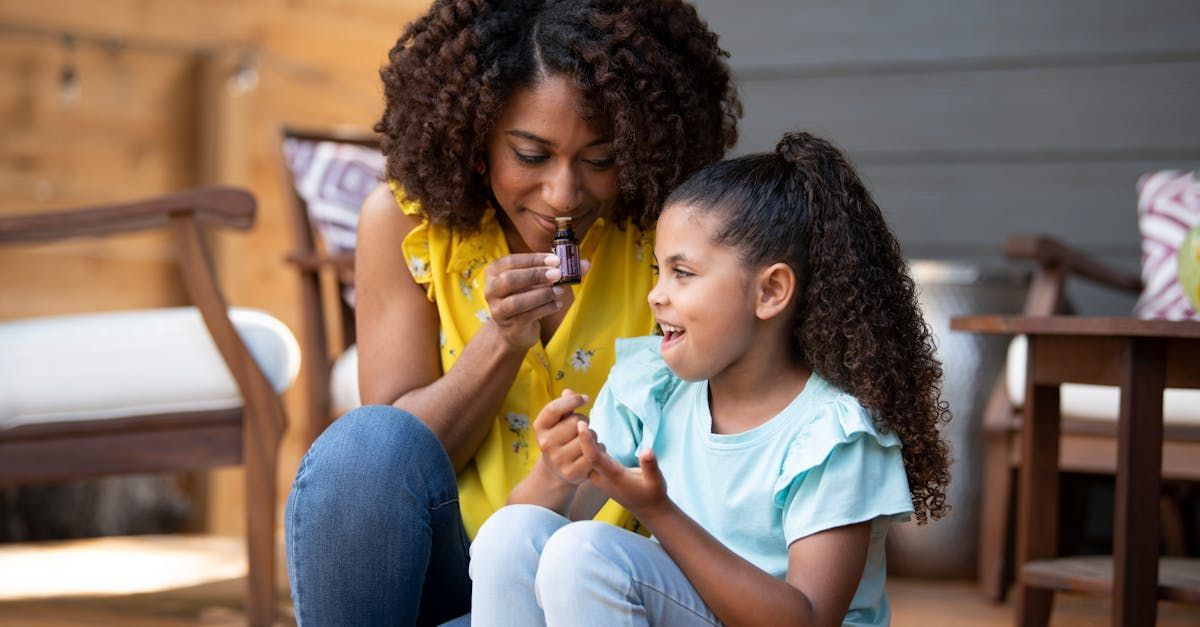
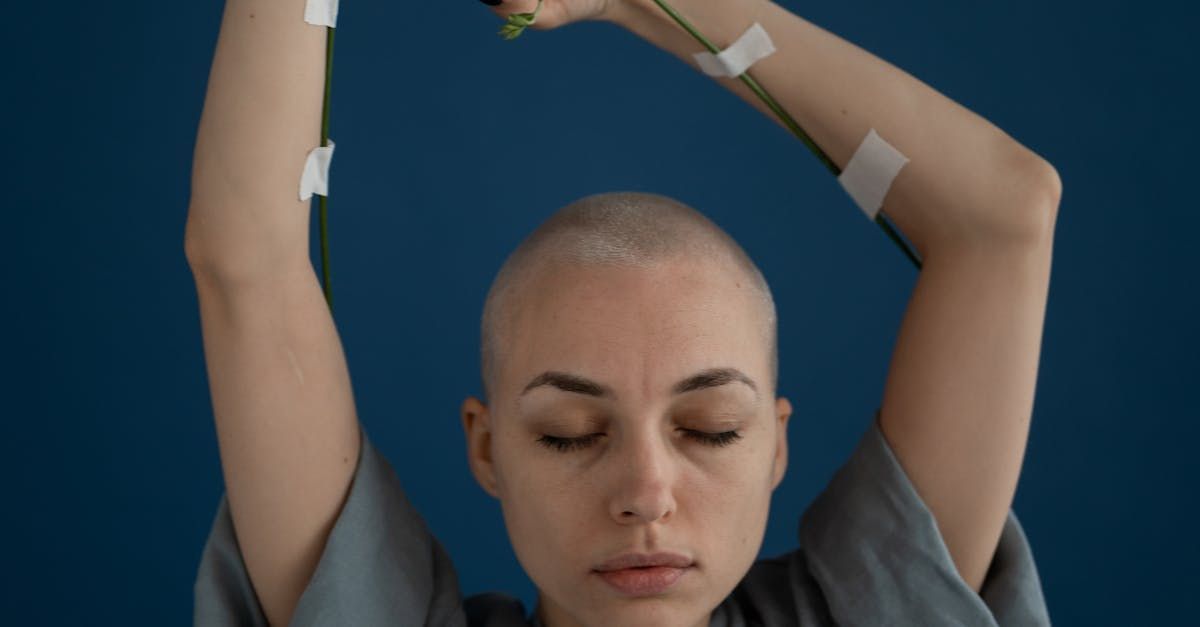
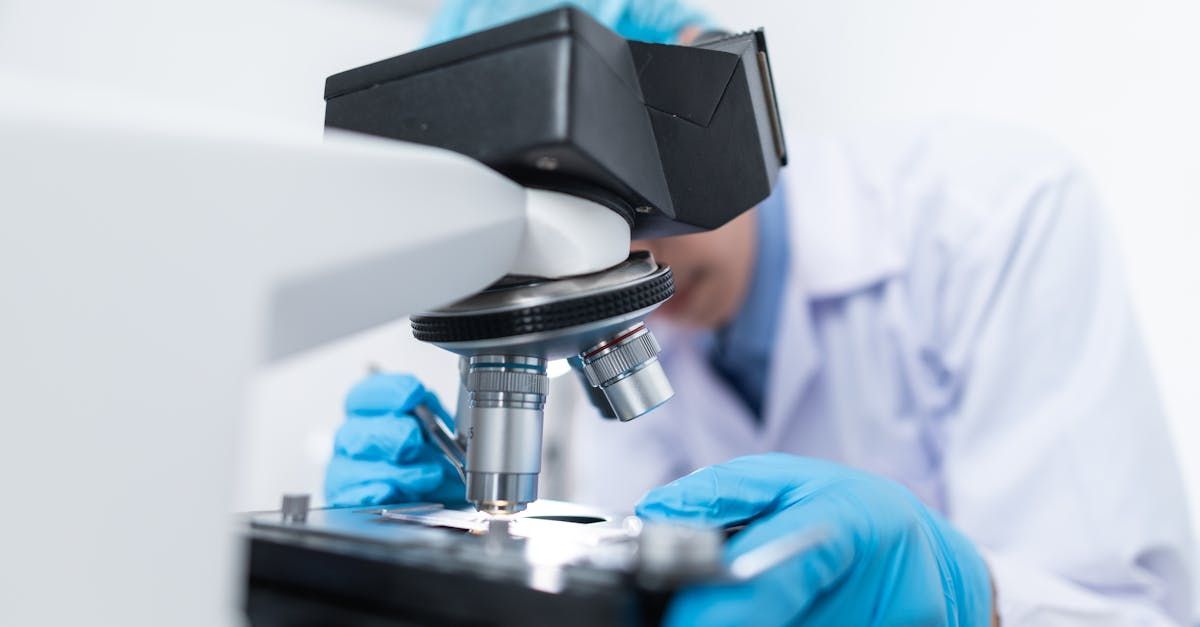
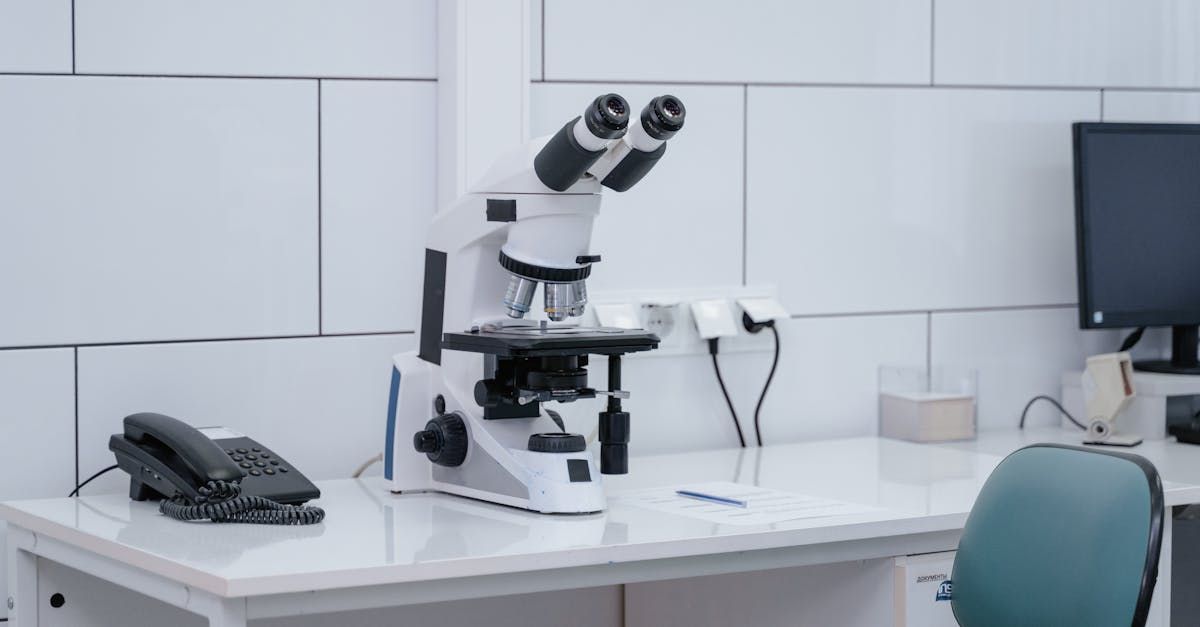
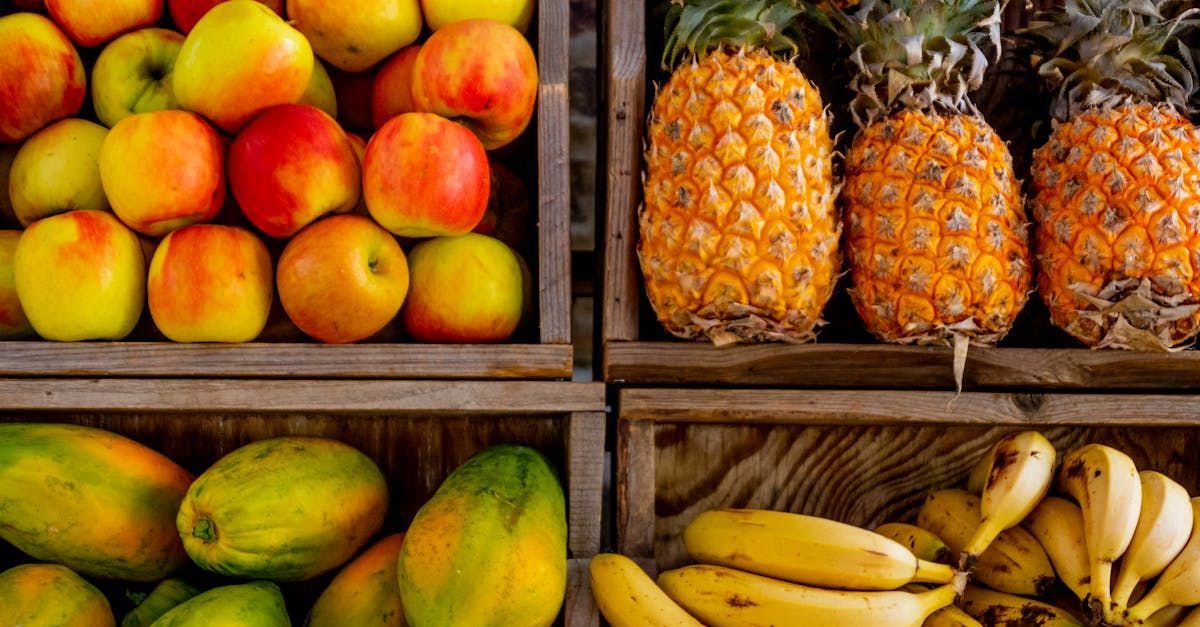
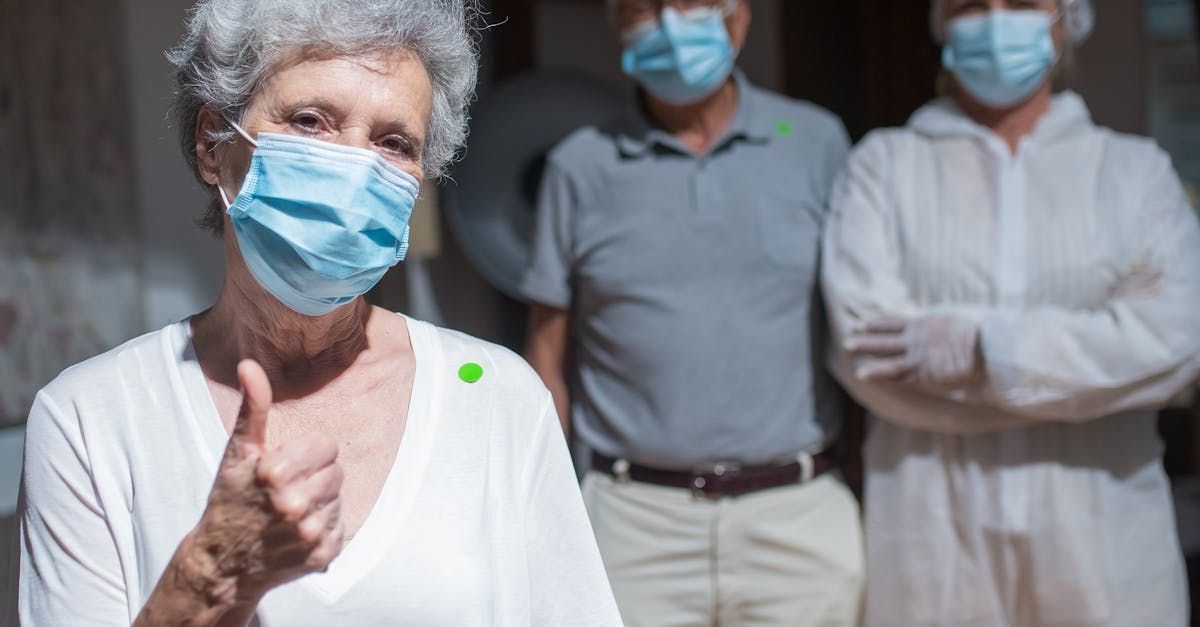
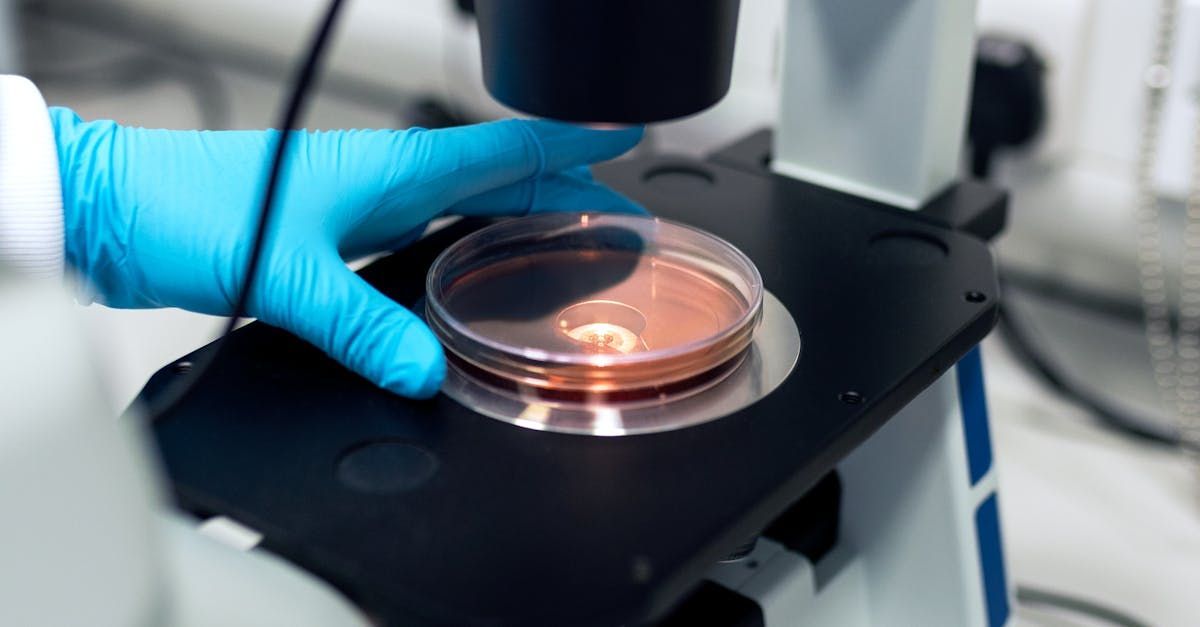
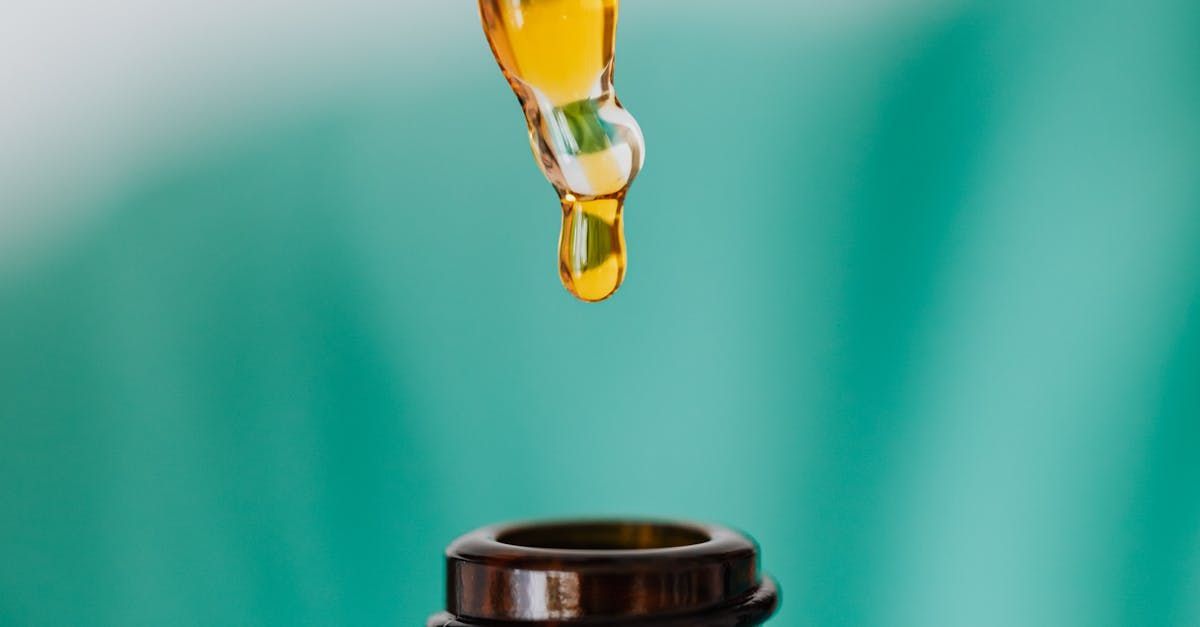
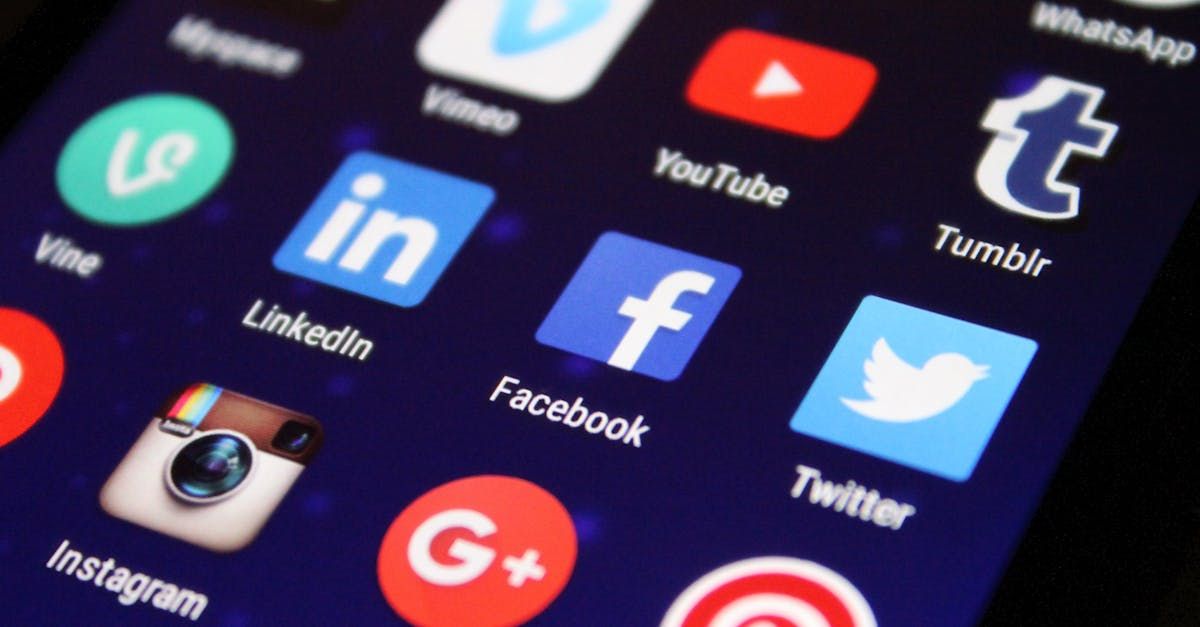