The Role of Artificial Intelligence in Drug Safety Monitoring
In the rapidly evolving landscape of healthcare, artificial intelligence (AI) has emerged as a transformative force, driving innovation across various domains. Among these, drug safety monitoring—also known as pharmacovigilance—stands out as a critical area where AI's potential is being increasingly recognized. Pharmacovigilance involves the continuous monitoring and assessment of adverse drug reactions (ADRs) to ensure patient safety. As the pharmaceutical industry grows and diversifies, the sheer volume and complexity of data generated pose significant challenges for traditional drug safety monitoring methods. This blog delves into the pivotal role AI plays in enhancing pharmacovigilance, exploring current challenges, practical applications, case studies, and future directions, while also addressing the ethical and regulatory considerations involved.
Current Challenges in Drug Safety Monitoring
Complexity and Volume of Data
The pharmaceutical industry generates an enormous amount of data, ranging from clinical trial results to patient-reported outcomes and spontaneous reports of ADRs. These data sources are often unstructured, diverse, and dispersed across various platforms, including electronic health records (EHRs), medical literature, and social media. The traditional methods of pharmacovigilance, which rely heavily on manual review and analysis, are increasingly inadequate to handle this data deluge. The complexity of integrating and analyzing such diverse data sources can lead to delays in identifying potential safety issues, thereby compromising patient safety.
Moreover, the globalization of the pharmaceutical market means that drugs are used in diverse populations, each with unique genetic, environmental, and behavioral characteristics. This diversity further complicates the detection of ADRs, as different populations may respond differently to the same medication. The need for more sophisticated tools to manage and analyze these vast and complex datasets is more pressing than ever.
Delays in Detecting Adverse Drug Reactions (ADRs)
One of the most critical issues in pharmacovigilance is the timely detection of ADRs. Traditional methods, such as spontaneous reporting systems and manual data analysis, often suffer from significant delays. Spontaneous reporting systems depend on healthcare professionals and patients to report adverse events, leading to underreporting and delays in the identification of safety signals. Manual analysis, on the other hand, is labor-intensive and time-consuming, further delaying the response to potential safety issues.
These delays can have serious consequences, as they may result in prolonged exposure of patients to harmful drugs. The thalidomide tragedy in the 1960s, which led to severe birth defects before the drug was withdrawn, is a stark reminder of the potential consequences of delayed ADR detection. While pharmacovigilance practices have improved since then, the need for faster and more efficient methods remains critical.
Limitations of Traditional Monitoring Methods
Traditional pharmacovigilance methods, including clinical trials and spontaneous reporting systems, have inherent limitations. Clinical trials, while rigorous, are conducted in controlled environments and often involve a relatively small number of participants. As a result, they may not capture rare ADRs or those that occur in specific subpopulations. Additionally, clinical trials are typically of limited duration, which means they may not detect long-term safety issues.
Spontaneous reporting systems, while valuable, are limited by underreporting and the quality of the data submitted. Reports may lack important details, such as patient demographics or concomitant medications, making it difficult to assess causality. Furthermore, these systems are often reactive rather than proactive, relying on the voluntary submission of reports rather than actively seeking out potential safety signals. Given these limitations, there is a clear need for more advanced, proactive approaches to drug safety monitoring.
AI Applications in Drug Safety Monitoring
Automated Signal Detection and Analysis
One of the most promising applications of AI in pharmacovigilance is automated signal detection and analysis. AI algorithms, particularly machine learning models, can be trained to sift through large datasets to identify patterns and correlations indicative of potential ADRs. These algorithms can process both structured data, such as clinical trial results and EHRs, and unstructured data, like social media posts and medical literature.
For example, natural language processing (NLP) techniques can be used to extract relevant information from textual data sources, identifying mentions of drug names, adverse events, and patient demographics. Machine learning models can then analyze this data to identify emerging safety signals that may not be immediately apparent through traditional methods. This automated approach allows for more rapid detection of potential safety issues, enabling quicker regulatory responses and potentially preventing harm to patients.
Predictive Modeling for ADRs
Predictive modeling is another area where AI shows great promise in drug safety monitoring. By analyzing historical data, machine learning models can identify patterns and risk factors associated with known ADRs. These models can then predict the likelihood of similar events occurring with new or existing drugs. This predictive capability is particularly valuable in the early stages of drug development, where it can help identify potential safety issues before a drug is widely used.
Predictive modeling can also be used to identify patient populations at higher risk of experiencing specific ADRs. For example, certain genetic markers or comorbid conditions may increase a patient's susceptibility to an adverse reaction. By identifying these risk factors, healthcare providers can make more informed decisions about prescribing medications, potentially reducing the incidence of ADRs. This personalized approach to medicine not only improves patient safety but also enhances the overall effectiveness of treatment.
Natural Language Processing for Data Extraction
NLP is a subset of AI that focuses on the interaction between computers and human language. In the context of pharmacovigilance, NLP techniques are used to process and analyze unstructured text data, such as clinical notes, research articles, and social media posts. This capability is crucial for extracting relevant information that may not be readily available in structured formats.
For example, NLP can be used to identify mentions of ADRs in social media posts or patient forums. This type of data is often unstructured and may contain informal language or slang, making it challenging to analyze using traditional methods. However, NLP algorithms can be trained to recognize specific terms and phrases, enabling the extraction of valuable insights from these data sources. This expanded scope of data analysis provides a more comprehensive view of drug safety, incorporating real-world evidence that may not be captured through conventional means.
Case Studies and Examples
Successful Implementations of AI in Pharmacovigilance
Several organizations and regulatory bodies have successfully implemented AI technologies in their pharmacovigilance efforts. One notable example is the U.S. Food and Drug Administration's (FDA) Sentinel Initiative. This system uses AI to monitor the safety of drugs and medical devices by analyzing data from EHRs, insurance claims, and other sources. The system's ability to process vast amounts of data quickly and accurately allows the FDA to detect potential safety signals more efficiently than traditional methods.
Another example is the European Medicines Agency's (EMA) EudraVigilance system, which collects and manages data on suspected ADRs. The EMA has incorporated AI algorithms into EudraVigilance to enhance the system's signal detection capabilities. These algorithms analyze both structured and unstructured data to identify potential safety issues. The successful integration of AI into these systems demonstrates the technology's potential to improve the efficiency and effectiveness of pharmacovigilance activities.
Impact on Drug Safety and Patient Outcomes
The integration of AI into pharmacovigilance has had a positive impact on drug safety and patient outcomes. One of the most significant benefits is the ability to detect ADRs more rapidly and accurately. This early detection allows regulatory bodies and healthcare providers to take timely action, such as updating drug labels, issuing warnings, or recalling unsafe medications. As a result, patients are less likely to be exposed to harmful drugs, and adverse events can be mitigated more effectively.
In addition to improving the detection of ADRs, AI-driven pharmacovigilance systems can also enhance patient care by providing more personalized treatment recommendations. For example, predictive models can identify patients at higher risk of experiencing specific ADRs, allowing healthcare providers to adjust treatment plans accordingly. This personalized approach not only improves patient safety but also enhances the overall effectiveness of treatment, leading to better health outcomes.
Lessons Learned and Insights
The implementation of AI in pharmacovigilance has provided valuable insights into best practices and potential challenges. One key lesson is the importance of data quality and integration. For AI systems to be effective, they require access to high-quality, diverse datasets. Ensuring that data is accurate, complete, and up-to-date is crucial for the success of AI-driven drug safety monitoring.
Additionally, organizations must consider the ethical implications of using AI in pharmacovigilance, particularly in terms of addressing potential biases and ensuring fairness in decision-making processes. For example, if the data used to train AI models is biased towards certain demographic groups, the resulting predictions may not accurately reflect the experiences of other groups. Addressing these biases and ensuring that AI models are trained on representative datasets is essential for promoting fairness and equity in pharmacovigilance.
Technological and Ethical Considerations
Data Quality and Integration
Data quality and integration are fundamental challenges in the deployment of AI for drug safety monitoring. High-quality data is critical for training accurate and reliable AI models. However, data quality issues, such as missing values, inconsistencies, and errors, can significantly impact the performance of these models. To address these issues, organizations must invest in robust data management practices, including data cleaning, standardization, and validation.
Data integration involves combining data from multiple sources, such as EHRs, clinical trials, and social media, to provide a comprehensive view of drug safety. This process can be challenging due to differences in data formats, standards, and privacy regulations. For example, integrating data from different countries may require navigating various legal and regulatory frameworks. Organizations must develop data governance frameworks and invest in the necessary infrastructure to ensure seamless data integration.
Addressing Bias and Ensuring Fairness
Bias in AI models is a significant concern in pharmacovigilance, as it can lead to unequal detection of ADRs among different patient populations. For example, if an AI model is trained primarily on data from clinical trials that predominantly include a specific demographic group, the model may be less effective at identifying ADRs in other groups. This issue highlights the importance of using diverse and representative datasets in training AI models.
To address bias and ensure fairness, organizations should implement measures to detect and mitigate biases in AI models. This may involve conducting regular audits of the models, using techniques such as fairness-aware machine learning, and incorporating feedback from diverse stakeholders. Additionally, transparency and explainability are crucial for ensuring that AI-driven decisions are understandable and accountable. Organizations should strive to make their AI systems as transparent as possible, providing clear explanations for the decisions made by the models.
Regulatory and Ethical Challenges
The use of AI in pharmacovigilance raises several regulatory and ethical challenges. From a regulatory perspective, there is a need for clear guidelines and standards for the development and deployment of AI systems in drug safety monitoring. These guidelines should address issues such as data privacy, model transparency, and accountability. Regulatory bodies must also ensure that AI-driven pharmacovigilance systems comply with existing laws and regulations, such as the General Data Protection Regulation (GDPR) in the European Union.
Ethically, organizations must consider the potential impact of AI-driven decisions on patient care and ensure that these decisions are made fairly and transparently. This includes addressing potential biases in AI models and ensuring that the data used to train these models is representative of the broader patient population. Additionally, organizations must navigate the complexities of data ownership and consent, particularly when using patient data for AI training and analysis. Ensuring that patients' rights and privacy are respected is crucial for maintaining public trust in AI-driven pharmacovigilance systems.
Future Directions and Innovations
Emerging AI Technologies in Drug Safety
The field of AI is continually evolving, with new technologies and methodologies emerging that could further enhance drug safety monitoring. One such technology is deep learning, a subset of machine learning that involves neural networks with many layers. Deep learning has shown promise in analyzing complex datasets and identifying subtle patterns indicative of ADRs. For example, deep learning models can analyze medical images, genomic data, and other high-dimensional data types, providing insights that may not be accessible through traditional methods.
Other emerging technologies, such as reinforcement learning and generative models, could also play a role in improving pharmacovigilance activities. Reinforcement learning involves training AI models to make decisions based on trial and error, which could be useful for optimizing treatment plans and minimizing the risk of ADRs. Generative models, on the other hand, can be used to simulate various scenarios, such as the potential impact of different drug dosages or combinations, providing valuable insights for drug development and safety monitoring.
Integration with Real-World Data and Electronic Health Records
The integration of AI with real-world data (RWD) and EHRs is a promising avenue for advancing drug safety monitoring. RWD includes data collected outside of traditional clinical trials, such as patient-reported outcomes, data from wearable devices, and social media posts. By incorporating RWD and EHRs into AI-driven pharmacovigilance systems, organizations can gain a more comprehensive understanding of drug safety in real-world settings.
For example, EHRs contain valuable information about patients' medical histories, comorbid conditions, and medication use. Integrating this data with AI-driven systems allows for more accurate detection of ADRs and better understanding of the factors that contribute to these events. Additionally, the use of RWD can help identify rare ADRs that may not be captured in clinical trials or spontaneous reporting systems. This integration also provides opportunities for continuous monitoring and real-time analysis, enabling more proactive and timely interventions.
Potential Impact on the Future of Pharmacovigilance
The continued advancement of AI technologies is likely to have a profound impact on the future of pharmacovigilance. AI-driven systems have the potential to transform drug safety monitoring from a reactive process to a proactive one. By enabling the early detection of safety signals and predicting potential ADRs, AI can help prevent adverse events before they occur. This proactive approach not only improves patient safety but also enhances the overall effectiveness of healthcare systems.
Furthermore, the integration of AI with other emerging technologies, such as blockchain and the Internet of Things (IoT), could further enhance the transparency and efficiency of pharmacovigilance activities.
Blockchain technology, for example, can provide a secure and immutable record of data, ensuring the integrity and traceability of pharmacovigilance data. IoT devices, such as wearable health monitors, can provide real-time data on patients' health status, allowing for continuous monitoring and timely interventions.
As these technologies continue to develop and mature, they are likely to play an increasingly important role in drug safety monitoring. The widespread adoption of AI in pharmacovigilance could lead to safer medications, more personalized treatments, and improved patient outcomes. However, realizing this potential will require ongoing collaboration and innovation among all stakeholders involved.
Collaboration and Implementation Strategies
Collaboration Between Stakeholders
The successful implementation of AI in drug safety monitoring requires collaboration between various stakeholders, including regulatory bodies, pharmaceutical companies, healthcare providers, and technology developers. These stakeholders must work together to establish common standards and best practices for the use of AI in pharmacovigilance. Additionally, they should engage in open dialogue to address potential challenges and share insights and experiences.
Collaboration is also essential for ensuring that AI systems are developed and deployed in a way that aligns with regulatory requirements and ethical considerations. For example, regulatory bodies can provide guidance on data privacy and model transparency, while pharmaceutical companies can contribute their expertise in drug development and safety monitoring. By working together, stakeholders can ensure that AI-driven pharmacovigilance systems are safe, effective, and ethical.
Best Practices for Implementing AI in Drug Safety Monitoring
Organizations looking to implement AI in their pharmacovigilance activities should follow best practices to ensure the success of their initiatives. These practices include:
- Investing in High-Quality Data: High-quality data is essential for training accurate and reliable AI models. Organizations should invest in robust data management practices, including data cleaning, standardization, and validation.
- Conducting Thorough Validation and Testing: AI models should be thoroughly validated and tested to ensure their accuracy and reliability. This includes conducting regular audits of the models and implementing measures to detect and mitigate biases.
- Establishing Clear Governance Frameworks: Organizations should establish clear governance frameworks for the development and deployment of AI systems. This includes defining roles and responsibilities, setting standards for data privacy and security, and ensuring transparency and accountability.
- Prioritizing Transparency and Explainability: Transparency and explainability are crucial for ensuring that AI-driven decisions are understandable and accountable. Organizations should strive to make their AI systems as transparent as possible, providing clear explanations for the decisions made by the models.
- Providing Ongoing Training and Education: Ongoing training and education are essential for ensuring that staff are equipped to work with AI technologies. This includes providing training on the basics of AI, as well as specific skills related to data management and model interpretation.
Overcoming Barriers to Adoption
While the potential benefits of AI in drug safety monitoring are significant, several barriers to adoption must be addressed. These barriers include concerns about data privacy and security, the complexity of integrating AI with existing systems, and the need for regulatory clarity.
To overcome these challenges, organizations should engage with regulatory bodies and other stakeholders to develop clear guidelines and standards. This includes addressing issues such as data privacy, model transparency, and accountability. Additionally, organizations should invest in the necessary infrastructure and resources to support the implementation of AI technologies. This may involve upgrading existing systems, developing new data management practices, and providing training and education for staff.
Finally, organizations should prioritize communication and engagement with patients and the public. Ensuring that patients understand how their data is being used and how AI-driven decisions may impact their care is crucial for maintaining public trust in AI-driven pharmacovigilance systems.
Notable Labs: Pioneering AI in Drug Safety Monitoring
In the rapidly evolving landscape of healthcare, artificial intelligence (AI) has emerged as a transformative force, particularly in drug safety monitoring, or pharmacovigilance. Notable Labs is at the forefront of this innovation, utilizing AI to continuously monitor and assess adverse drug reactions (ADRs) to ensure patient safety. The pharmaceutical industry faces challenges from the sheer volume and complexity of data generated, including clinical trial results, patient-reported outcomes, and spontaneous ADR reports. Traditional monitoring methods struggle with this data deluge, often leading to delays in identifying potential safety issues.
Notable Labs addresses these challenges by leveraging advanced AI technologies. Their systems can analyze vast datasets from diverse sources, including electronic health records (EHRs) and social media, to detect ADRs more quickly and accurately than traditional methods. By using predictive modeling and natural language processing (NLP), Notable Labs not only identifies emerging safety signals but also predicts potential ADRs, helping to prevent adverse events before they occur.
This proactive approach is vital as the pharmaceutical market becomes increasingly global, with drugs used across diverse populations. Notable Labs' AI-driven systems are designed to be inclusive and fair, ensuring that data from all demographic groups is considered, thus mitigating bias and promoting equity in pharmacovigilance. Their work exemplifies the potential of AI to transform drug safety monitoring, making it more efficient, comprehensive, and responsive to real-world data. Through continuous innovation and collaboration with stakeholders, Notable Labs is helping to shape the future of pharmacovigilance, ultimately leading to safer medications and improved patient outcomes.
Conclusion
The integration of artificial intelligence into drug safety monitoring represents a significant step forward in the field of pharmacovigilance. AI has the potential to enhance the detection and analysis of adverse drug reactions, predict potential safety issues, and provide valuable insights into real-world drug safety. While there are challenges to overcome, including data quality, bias, and regulatory considerations, the benefits of AI-driven pharmacovigilance are clear. By embracing AI technologies, stakeholders in the pharmaceutical industry can improve patient safety and outcomes.
As the field continues to evolve, collaboration and innovation will be key to realizing the full potential of AI in drug safety monitoring. Stakeholders must work together to establish common standards and best practices, address regulatory and ethical challenges, and ensure that AI systems are transparent, accountable, and fair. With the right strategies in place, AI has the potential to transform drug safety monitoring, leading to safer medications, more personalized treatments, and improved patient outcomes. The future of pharmacovigilance is bright, and AI will undoubtedly play a central role in shaping this future.
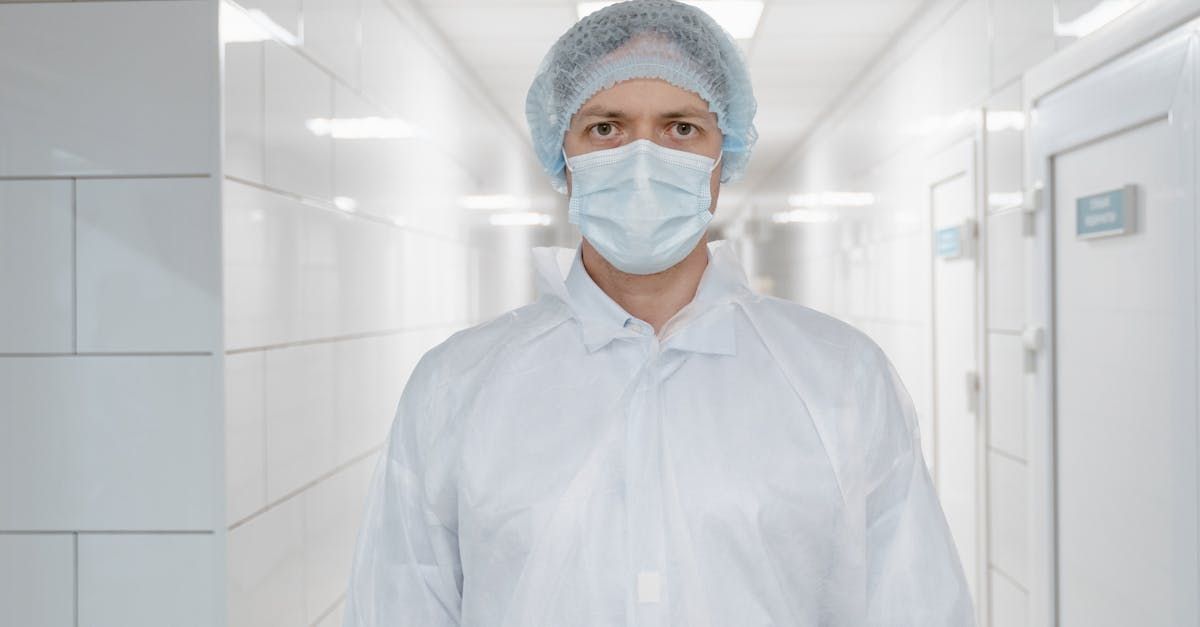
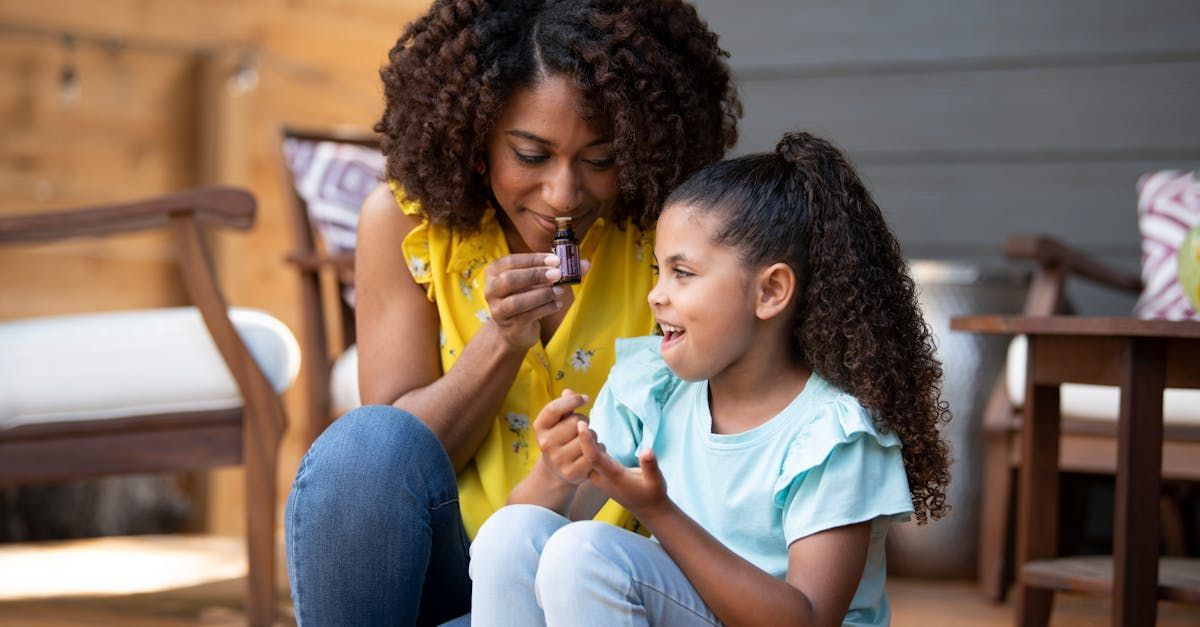
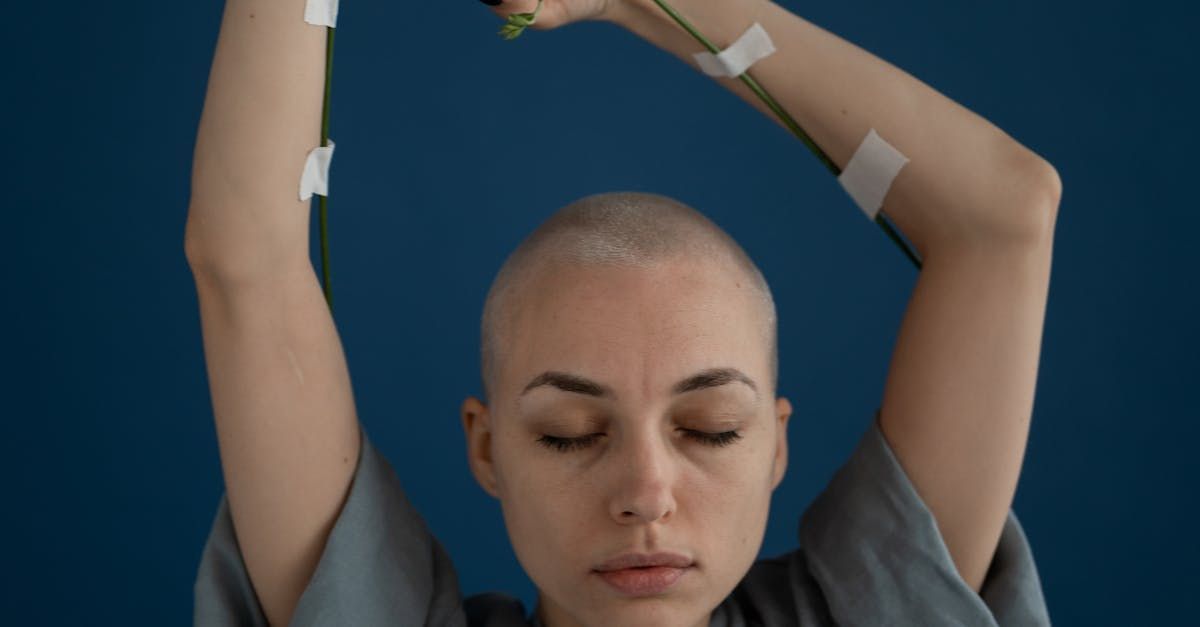
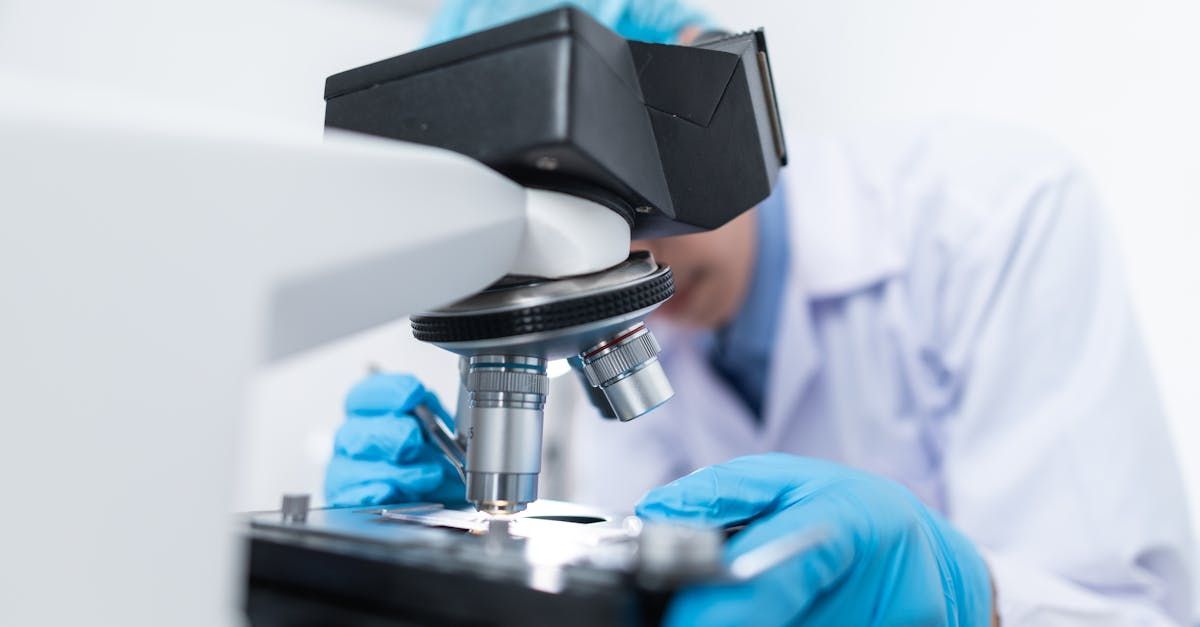
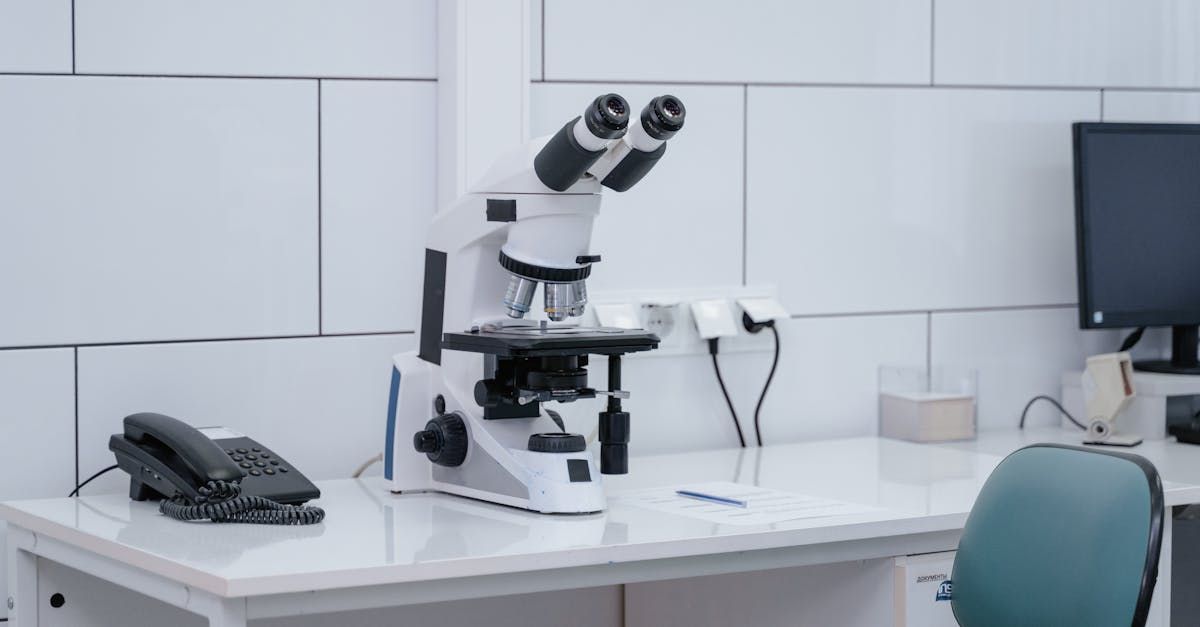
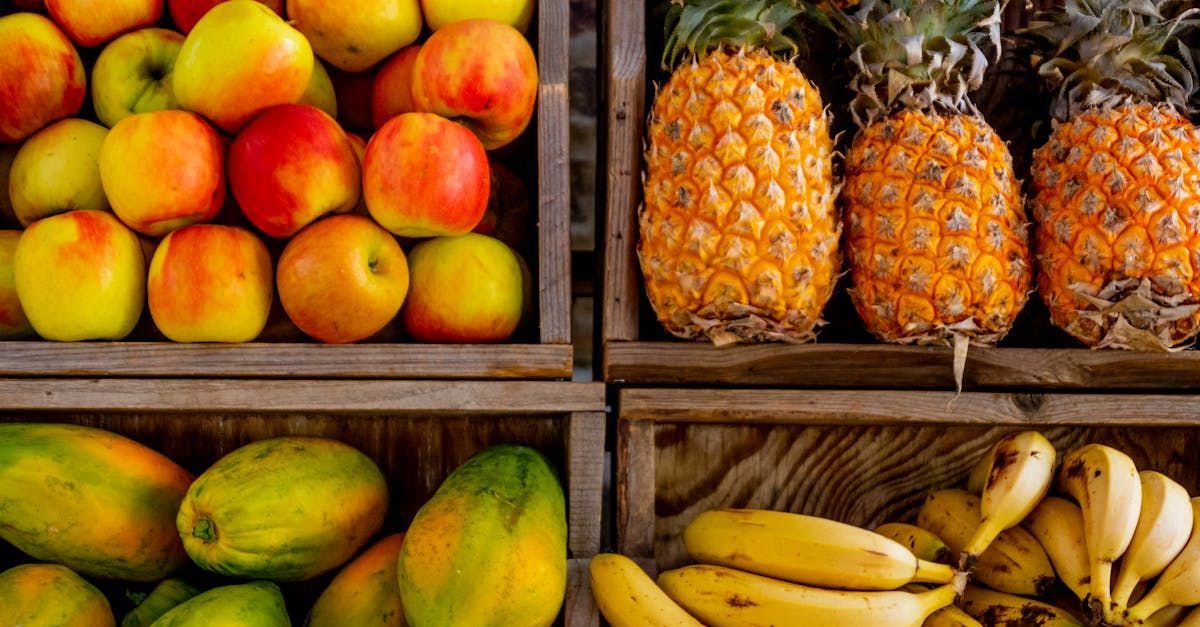
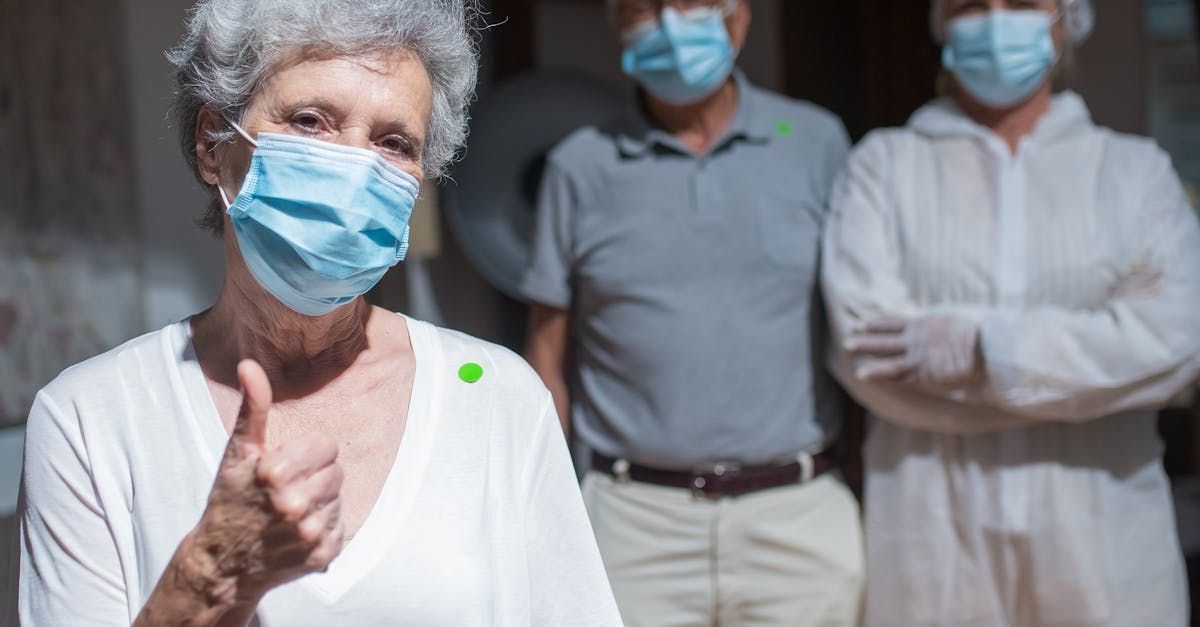
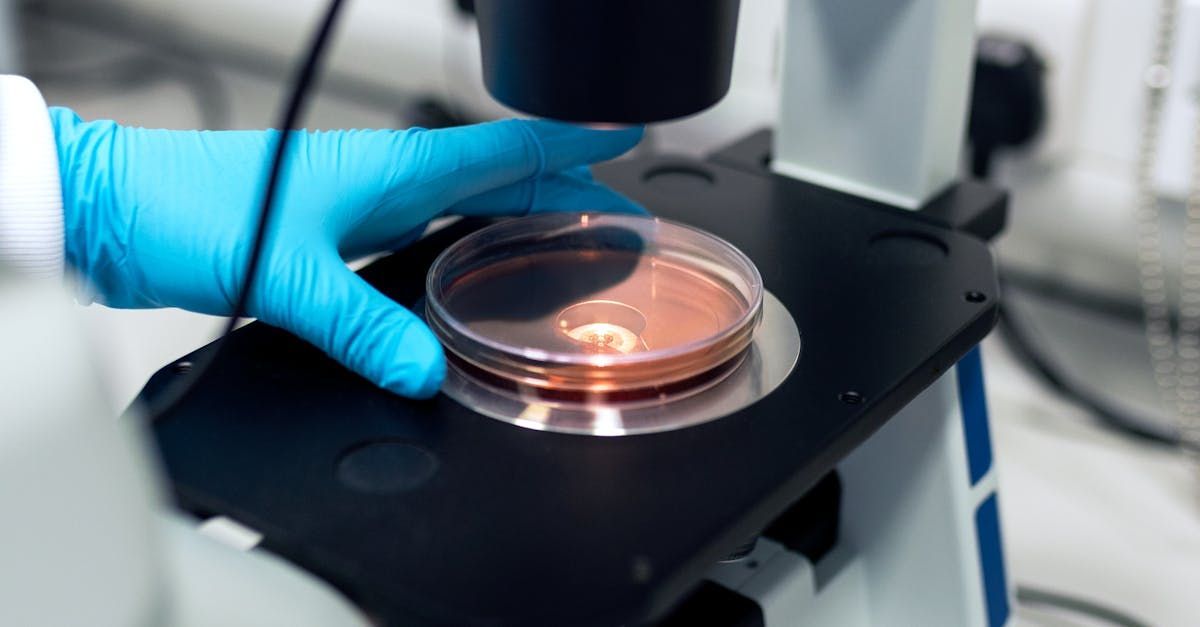
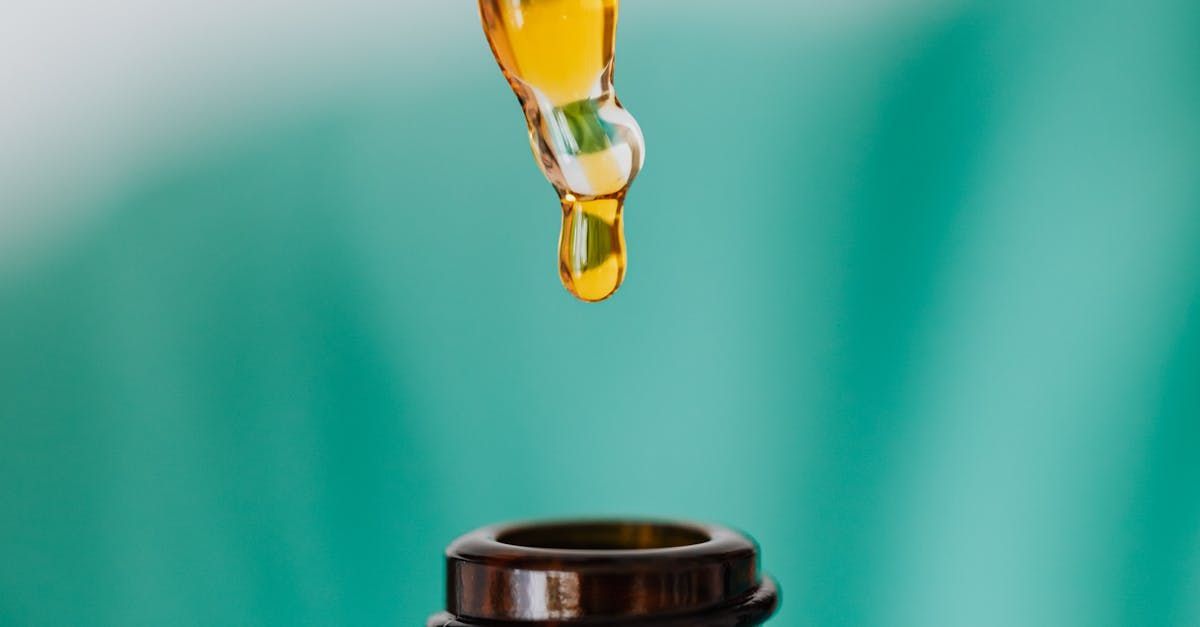
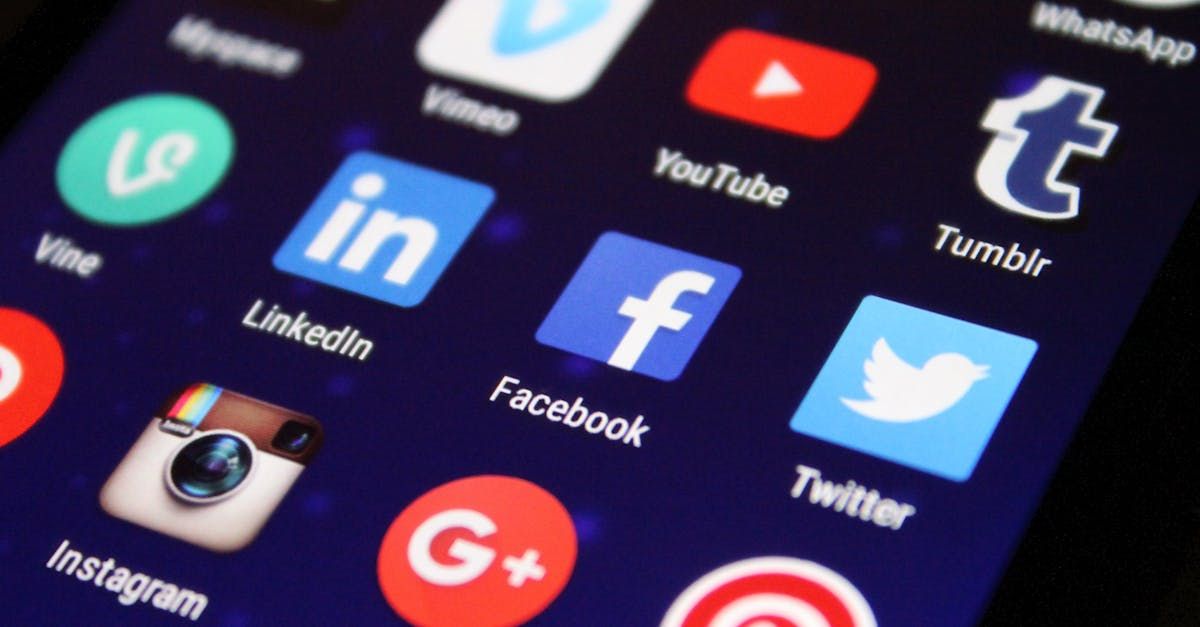