AI and Machine Learning in Clinical Research: Transforming Data-Driven Decision Making
In the rapidly evolving landscape of clinical research, the integration of artificial intelligence (AI) and machine learning (ML) technologies is poised to revolutionize the way we approach data analysis, decision-making, and ultimately, patient care. As the volume and complexity of biomedical data continue to grow exponentially, traditional analytical methods are increasingly challenged to extract meaningful insights and patterns. AI and ML offer powerful tools to harness the vast potential of this data, enabling more accurate predictions, personalized treatment strategies, and accelerated drug development processes.
Defining AI and Machine Learning in Clinical Research
Artificial Intelligence (AI) refers to the development of computer systems capable of performing tasks that typically require human intelligence, such as learning, reasoning, problem-solving, and decision-making. In the context of clinical research, AI encompasses a broad range of techniques and algorithms designed to analyze and interpret complex biomedical data.
Machine Learning (ML), a subset of AI, focuses on the development of algorithms and statistical models that enable systems to learn from data and improve their performance on specific tasks without being explicitly programmed. ML algorithms can identify patterns, make predictions, and adapt their decision-making processes based on the input data, making them particularly valuable in clinical research applications.
The integration of AI and ML in clinical research has the potential to transform various aspects of the drug development pipeline, from target identification and biomarker discovery to clinical trial design and patient stratification. By leveraging the power of these technologies, researchers can unlock new insights, optimize processes, and ultimately accelerate the delivery of innovative and personalized therapies to patients.
Applications of AI and Machine Learning in Clinical Research
The applications of AI and ML in clinical research are vast and continuously expanding. Here are some key areas where these technologies are making a significant impact:
Biomarker Discovery and Target Identification
AI and ML algorithms can analyze vast amounts of genomic, proteomic, and other omics data to identify potential biomarkers and therapeutic targets. By uncovering complex patterns and relationships within these datasets, researchers can gain valuable insights into disease mechanisms and develop more targeted and effective treatments.
Drug Discovery and Repurposing
AI and ML techniques can be applied to virtual screening of chemical libraries, molecular docking simulations, and predictive modeling of drug-target interactions. These approaches can accelerate the identification of promising drug candidates and facilitate the repurposing of existing drugs for new therapeutic indications, reducing the time and cost associated with traditional drug discovery processes.
Clinical Trial Design and Patient Recruitment
AI and ML algorithms can analyze real-world data from electronic health records (EHRs), patient registries, and other sources to optimize clinical trial design and patient recruitment strategies. By identifying eligible patient populations, stratifying risk factors, and predicting potential trial outcomes, these technologies can improve the efficiency and success rates of clinical trials.
Patient Stratification and Precision Medicine
AI and ML techniques can be used to analyze multi-omics data, clinical data, and patient-reported outcomes to stratify patient populations based on their molecular profiles, disease subtypes, and predicted treatment responses. This approach enables the development of personalized treatment strategies, improving patient outcomes and reducing the risk of adverse events.
Clinical Decision Support Systems
AI-powered clinical decision support systems (CDSS) can assist healthcare professionals in making informed decisions by analyzing patient data, treatment guidelines, and evidence-based recommendations. These systems can provide real-time guidance on diagnosis, treatment selection, and risk assessment, improving the quality and consistency of care delivery.
Medical Imaging Analysis
AI and ML algorithms have demonstrated remarkable capabilities in analyzing medical imaging data, such as X-rays, CT scans, and MRI images. These technologies can assist in disease detection, lesion segmentation, and quantitative analysis, enhancing diagnostic accuracy and enabling earlier intervention.
Natural Language Processing (NLP)
NLP techniques, a branch of AI, can be applied to analyze unstructured clinical data, such as physician notes, research literature, and patient narratives. By extracting valuable information from these text-based sources, NLP can support clinical decision-making, literature review processes, and the generation of new hypotheses for further investigation.
Predictive Modeling and Risk Stratification
AI and ML algorithms can be trained on large datasets to develop predictive models for various clinical outcomes, such as disease progression, treatment response, and adverse event risk. These models can inform clinical decision-making, enable early intervention strategies, and support personalized care plans for individual patients.
Clinical Trial Monitoring and Data Quality Assurance
AI and ML techniques can be employed to monitor clinical trial data in real-time, identifying potential data quality issues, protocol deviations, and safety signals. This proactive approach can enhance data integrity, ensure patient safety, and improve the overall quality of clinical research.
Pharmacovigilance and Post-Marketing Surveillance
AI and ML algorithms can analyze large volumes of post-marketing data, including adverse event reports, electronic health records, and social media data, to identify potential safety signals and adverse drug reactions. This can support proactive risk management strategies and inform regulatory decision-making processes.
Challenges and Considerations in Adopting AI and ML in Clinical Research
While the potential benefits of AI and ML in clinical research are significant, their adoption and implementation present several challenges and considerations:
Data Quality and Availability
The performance of AI and ML algorithms heavily relies on the quality and availability of training data. Ensuring the accuracy, completeness, and representativeness of biomedical data is crucial for developing reliable and unbiased models. Addressing data quality issues, such as missing values, inconsistencies, and biases, is a critical step in the successful implementation of these technologies.
Interpretability and Transparency
Many AI and ML models, particularly deep learning algorithms, can be perceived as "black boxes," making it challenging to understand and interpret their decision-making processes. Ensuring transparency and interpretability is essential for building trust in these systems, particularly in high-stakes clinical applications where decisions can have significant implications for patient care.
Regulatory and Ethical Considerations
The integration of AI and ML in clinical research raises important regulatory and ethical considerations. Ensuring patient privacy, data security, and algorithmic fairness are crucial aspects that must be addressed. Regulatory frameworks and guidelines need to evolve to accommodate the unique challenges posed by these technologies, while maintaining rigorous standards for patient safety and ethical conduct.
Validation and Reproducibility
Validating the performance and reproducibility of AI and ML models in clinical settings is a critical challenge. Robust validation strategies, including external validation on independent datasets, are necessary to ensure the generalizability and reliability of these models across diverse patient populations and healthcare settings.
Interdisciplinary Collaboration
Effective implementation of AI and ML in clinical research requires close collaboration among diverse stakeholders, including clinicians, researchers, data scientists, and regulatory bodies. Fostering interdisciplinary communication, knowledge sharing, and a common understanding of the capabilities and limitations of these technologies is essential for their successful adoption and integration into clinical practice.
Workforce Development and Training
As AI and ML technologies continue to evolve, there is a growing need for a skilled workforce capable of developing, deploying, and interpreting these advanced analytical tools. Investing in workforce development, specialized training programs, and continuous education initiatives is crucial to ensure the effective utilization of AI and ML in clinical research.
Computational Resources and Infrastructure
The development and deployment of AI and ML models often require significant computational resources, including high-performance computing infrastructure, specialized hardware (e.g., graphics processing units), and scalable data storage and processing capabilities. Ensuring access to these resources and establishing robust computational infrastructures is a prerequisite for leveraging the full potential of these technologies in clinical research.
Continuous Learning and Adaptation
AI and ML models need to be continuously updated and adapted as new data becomes available and as clinical practices evolve. Establishing mechanisms for continuous learning, model retraining, and iterative improvement is essential to maintain the relevance and accuracy of these models over time.
Integration with Existing Systems and Workflows
Seamless integration of AI and ML solutions into existing clinical research workflows and healthcare systems is a significant challenge. Addressing interoperability issues, ensuring compatibility with legacy systems, and minimizing disruptions to established processes are crucial for successful adoption and user acceptance.
Public Trust and Acceptance
Building public trust and acceptance of AI and ML technologies in clinical research is paramount. Addressing concerns related to privacy, bias, and the potential for unintended consequences is essential for fostering widespread adoption and ensuring that these technologies are leveraged in an ethical and responsible manner.
Despite these challenges, the potential of AI and ML in clinical research is undeniable.
By addressing these considerations and fostering collaborative efforts among stakeholders, the healthcare ecosystem can harness the power of these technologies to drive innovation, improve patient outcomes, and advance the frontiers of biomedical research.
Future Directions and Emerging Trends
The field of AI and ML in clinical research is rapidly evolving, with emerging trends and innovations shaping its future trajectory:
Federated Learning and Privacy-Preserving AI
Federated learning and privacy-preserving AI techniques enable the training of AI models on decentralized data sources while preserving data privacy and security. These approaches can facilitate collaborative model development and knowledge sharing across healthcare institutions, without the need for centralized data pooling.
Explainable AI (XAI)
Explainable AI (XAI) focuses on developing AI models that are interpretable and transparent, providing insights into their decision-making processes. XAI techniques can enhance trust and acceptance of AI systems in clinical settings, enabling clinicians and researchers to understand the rationale behind model predictions and recommendations.
Multimodal Learning and Data Fusion
Multimodal learning approaches combine and integrate diverse data modalities, such as imaging, genomics, clinical notes, and wearable sensor data, to develop more comprehensive and accurate AI models. Data fusion techniques can unlock new insights by leveraging the complementary information present in different data sources.
Reinforcement Learning and Sequential Decision-Making
Reinforcement learning algorithms can be applied to sequential decision-making problems in clinical research, such as optimizing treatment regimens, adaptive clinical trial designs, and personalized care pathways. These techniques can learn from experience and adapt to dynamic environments, enabling more effective and personalized decision-making processes.
Generative AI and Synthetic Data Generation
Generative AI models, such as generative adversarial networks (GANs) and variational autoencoders (VAEs), can be used to generate synthetic biomedical data, including images, omics data, and patient records. These techniques can augment existing datasets, enabling more robust model training and addressing data scarcity challenges.
Causal Inference and Counterfactual Reasoning
Causal inference and counterfactual reasoning techniques aim to uncover causal relationships and estimate the potential outcomes of interventions or treatments. These approaches can provide valuable insights into treatment effects, enable personalized decision-making, and support the development of more effective interventions.
AI-Assisted Drug Design and Development
AI and ML techniques are increasingly being applied to various stages of the drug development pipeline, from virtual screening and molecular modeling to clinical trial optimization and pharmacovigilance. AI-assisted drug design and development approaches have the potential to accelerate the discovery and development of novel therapeutics.
Real-World Evidence and Digital Biomarkers
The integration of real-world evidence (RWE) and digital biomarkers derived from wearable devices, mobile apps, and remote monitoring technologies can enhance the accuracy and relevance of AI models in clinical research. By leveraging these data sources, AI systems can better capture real-world patient experiences and outcomes.
Collaborative AI Platforms and Ecosystems
The development of collaborative AI platforms and ecosystems can facilitate knowledge sharing, model exchange, and collaborative model development among researchers, clinicians, and industry partners. These platforms can foster interdisciplinary collaboration, accelerate innovation, and enable the translation of AI-driven insights into clinical practice.
Regulatory Frameworks and Governance
As the adoption of AI and ML in clinical research continues to grow, there is a need for robust regulatory frameworks and governance mechanisms to ensure the safe, ethical, and responsible use of these technologies. Ongoing efforts to develop guidelines, standards, and best practices will be crucial for maintaining public trust and enabling the widespread adoption of AI and ML in healthcare.
The integration of AI and ML in clinical research represents a paradigm shift in how we approach biomedical data analysis, decision-making, and ultimately, patient care. By harnessing the power of these technologies, researchers and clinicians can unlock new insights, optimize processes, and accelerate the delivery of innovative and personalized therapies. However, realizing the full potential of AI and ML in clinical research requires a collaborative effort among stakeholders, addressing challenges related to data quality, interpretability, regulatory considerations, and workforce development. As the field continues to evolve, emerging trends and innovations will shape the future trajectory of AI and ML in clinical research, driving transformative advancements in healthcare and improving patient outcomes on a global scale.
Notable Labs' Use of AI and Machine Learning in Clinical Research
At Notable Labs, the integration of artificial intelligence (AI) and machine learning (ML) technologies is transforming clinical research by enhancing data analysis, decision-making, and patient care. By leveraging AI and ML, Notable Labs can process vast and complex datasets to identify patterns, make predictions, and develop personalized treatment strategies. These technologies enable the company to streamline the drug development process, optimize clinical trial designs, and improve patient outcomes.
One of the key applications of AI and ML at Notable Labs is in patient stratification and precision medicine. By analyzing multi-omics data, clinical records, and patient-reported outcomes, Notable Labs can classify patients into subgroups based on their molecular profiles and predicted responses to treatments. This allows for the development of tailored therapeutic approaches, ensuring that patients receive the most effective and personalized care. Additionally, AI-powered predictive models help in identifying potential adverse events and optimizing treatment plans, thereby enhancing the overall efficacy and safety of clinical trials. Through these innovative applications, Notable Labs is at the forefront of utilizing AI and ML to drive advancements in precision medicine and improve patient outcomes.
Conclusion
The integration of AI and machine learning in clinical research represents a transformative shift in the way we analyze data, make decisions, and ultimately, care for patients. These advanced technologies offer powerful tools to handle the growing volume and complexity of biomedical data, enabling more accurate predictions, personalized treatment strategies, and accelerated drug development processes. From biomarker discovery and drug repurposing to clinical trial design and patient stratification, AI and ML are revolutionizing various aspects of the drug development pipeline, driving innovation and improving patient outcomes.
Despite the significant potential of AI and ML, their adoption in clinical research comes with challenges, including data quality, interpretability, regulatory considerations, and the need for interdisciplinary collaboration. Addressing these challenges through robust validation strategies, transparent regulatory frameworks, and continuous learning will be crucial for realizing the full potential of these technologies. As the field continues to evolve, emerging trends such as federated learning, explainable AI, and collaborative AI platforms will shape the future trajectory of AI and ML in clinical research, fostering transformative advancements in healthcare.
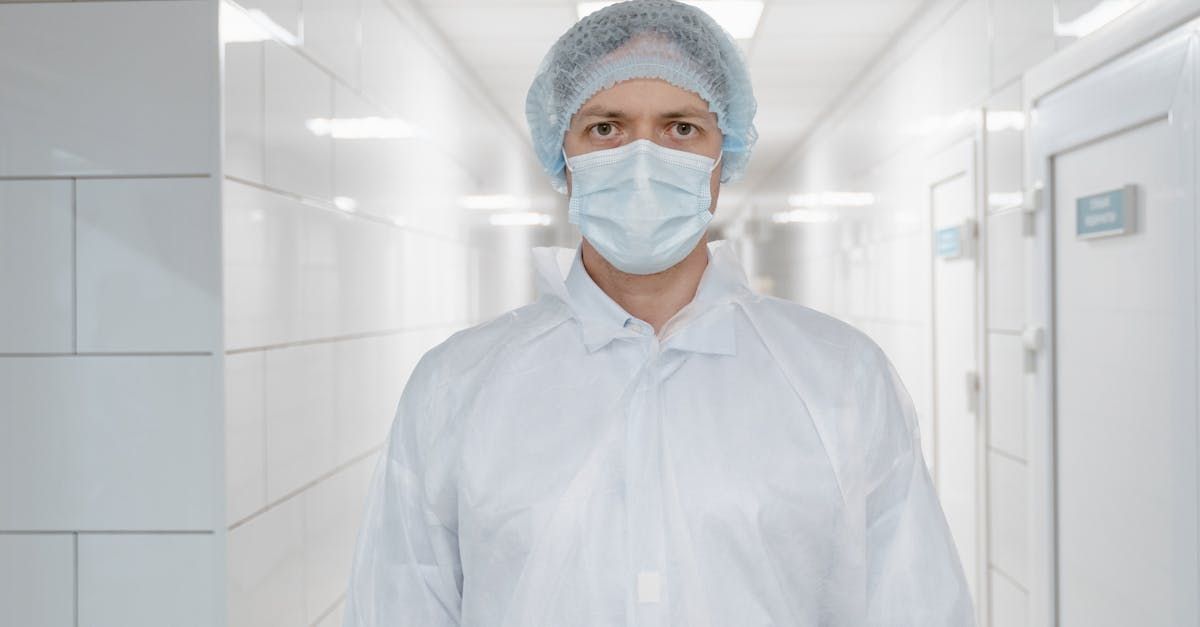
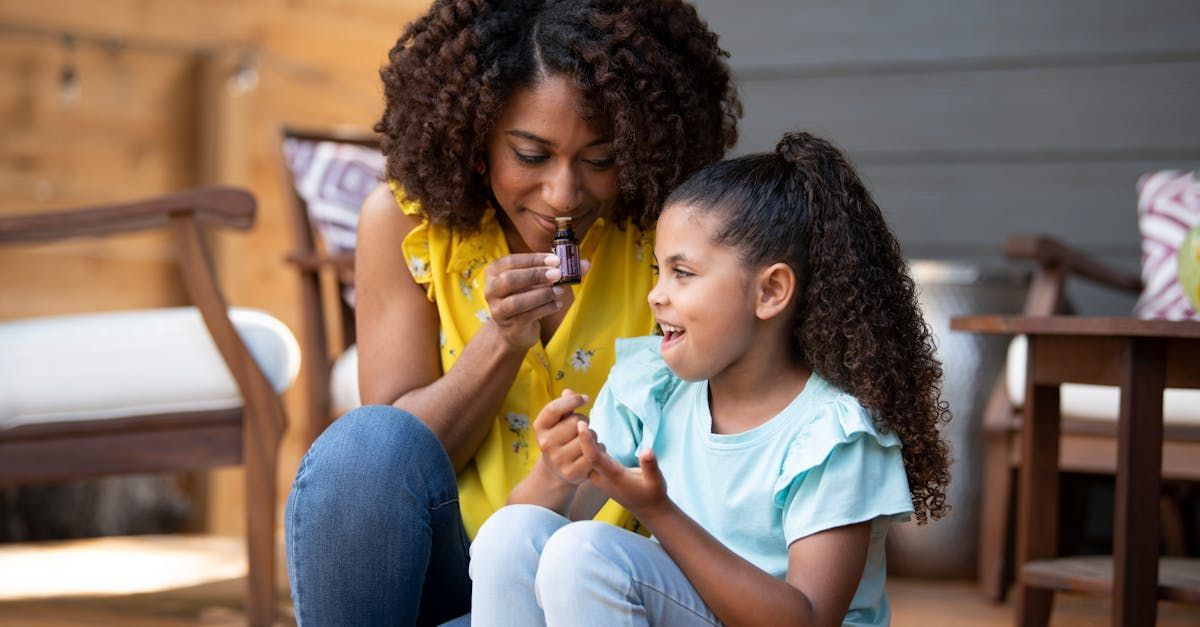
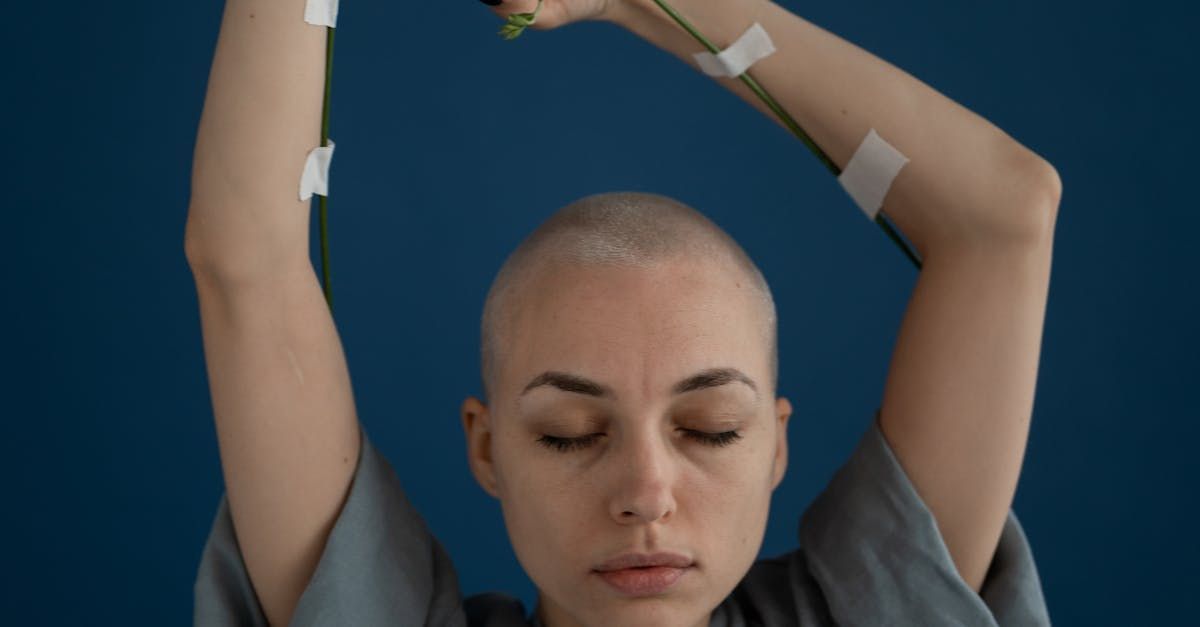
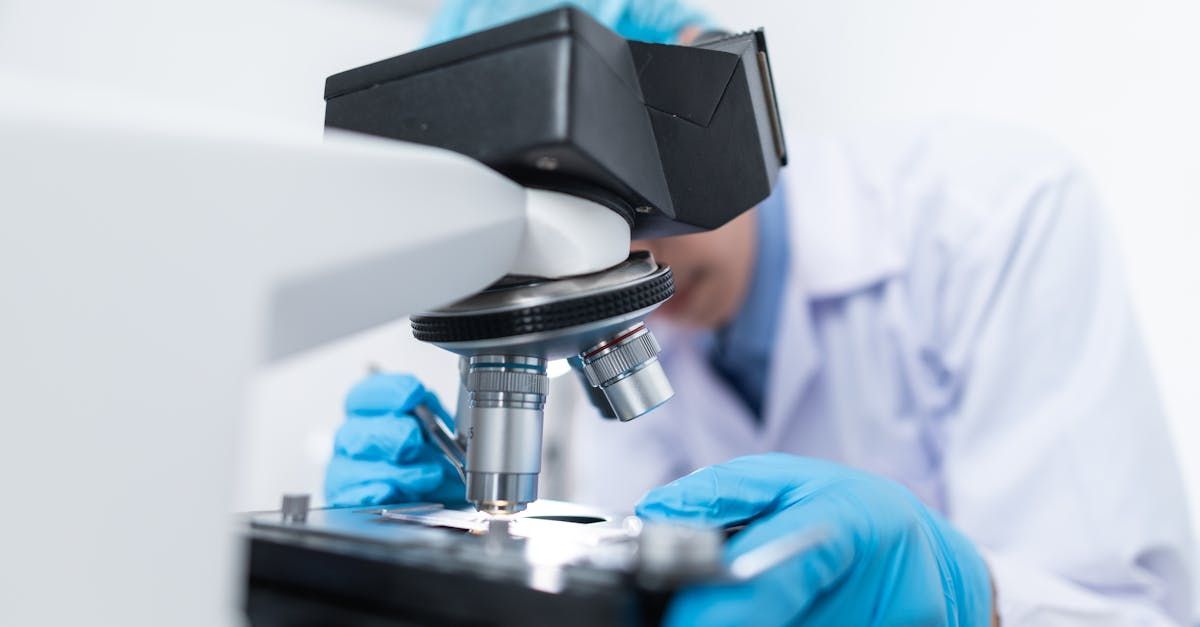
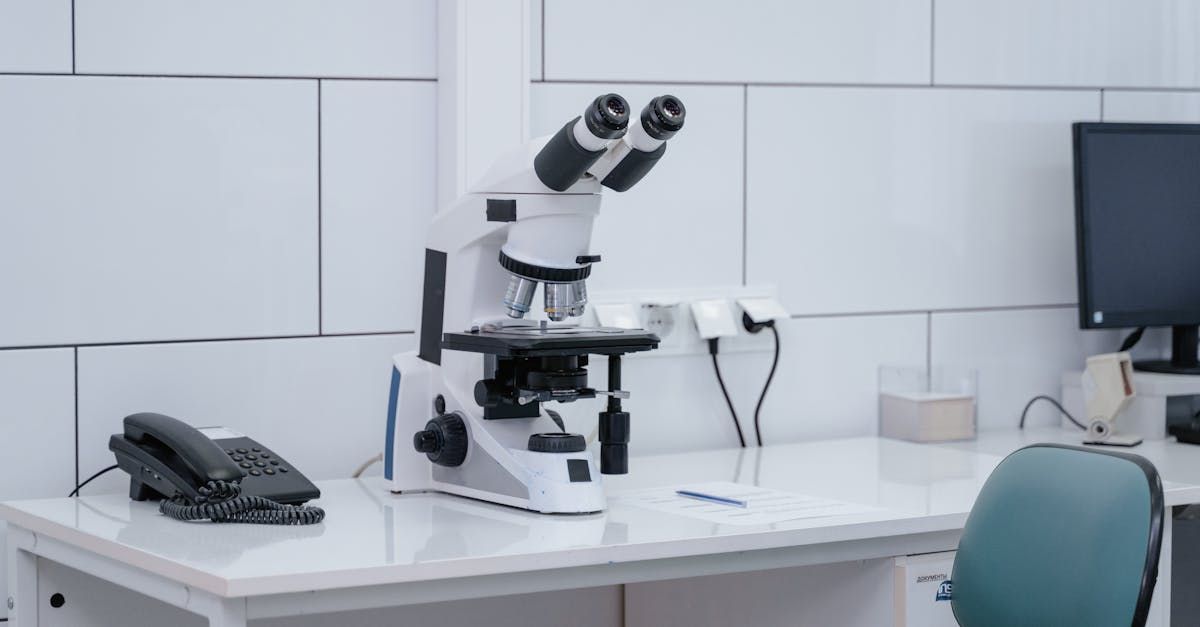
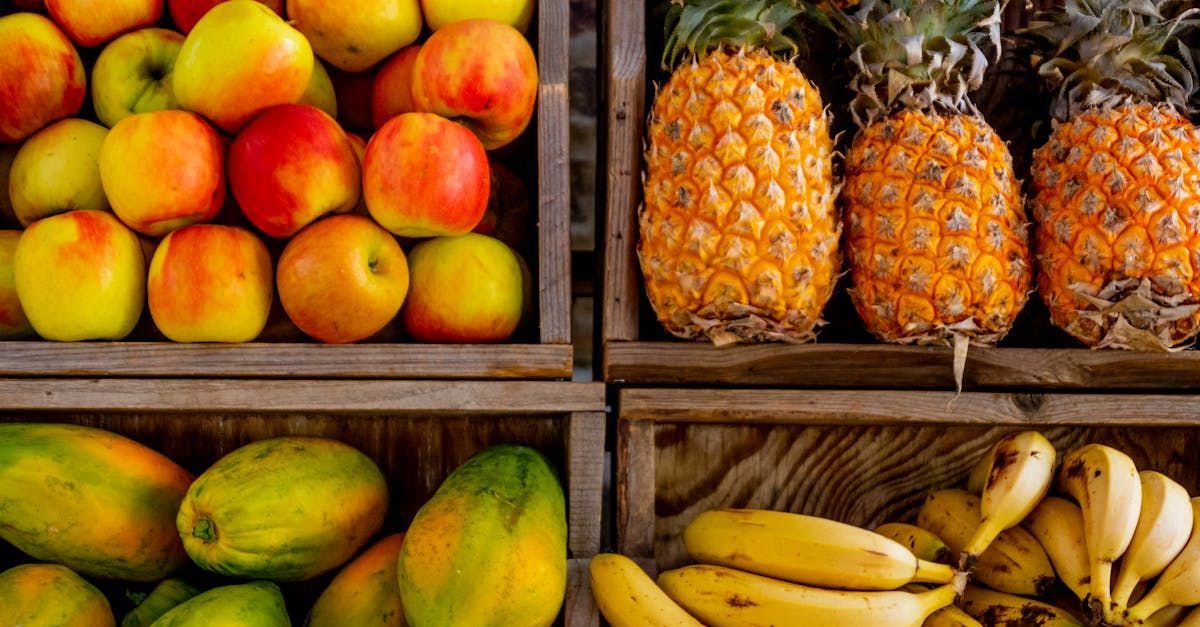
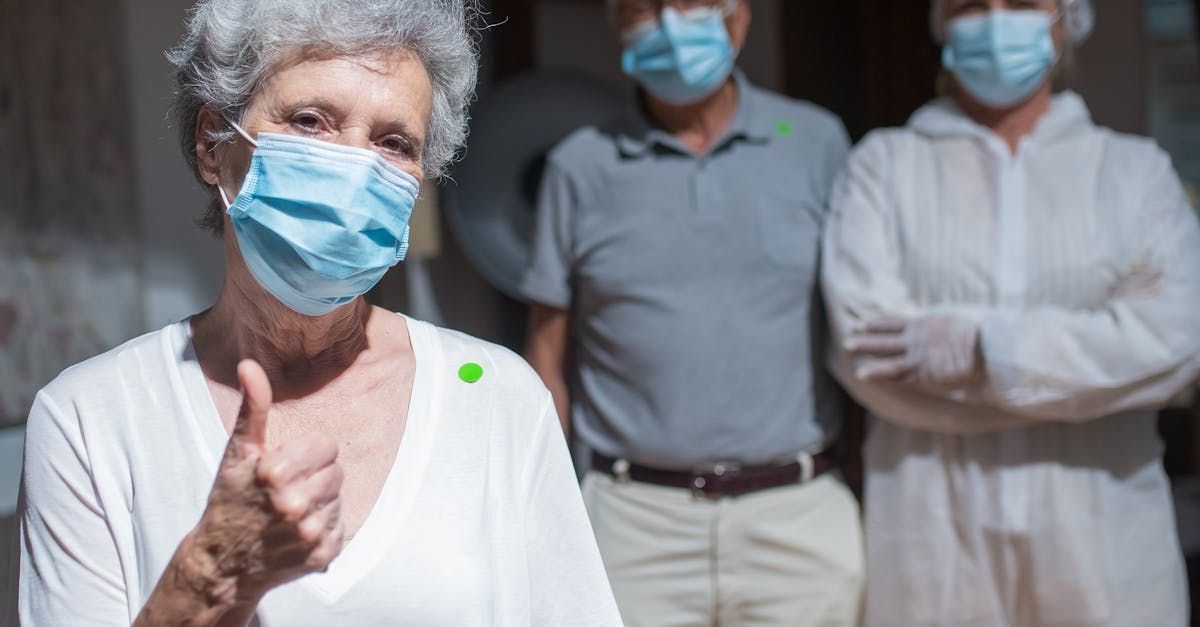
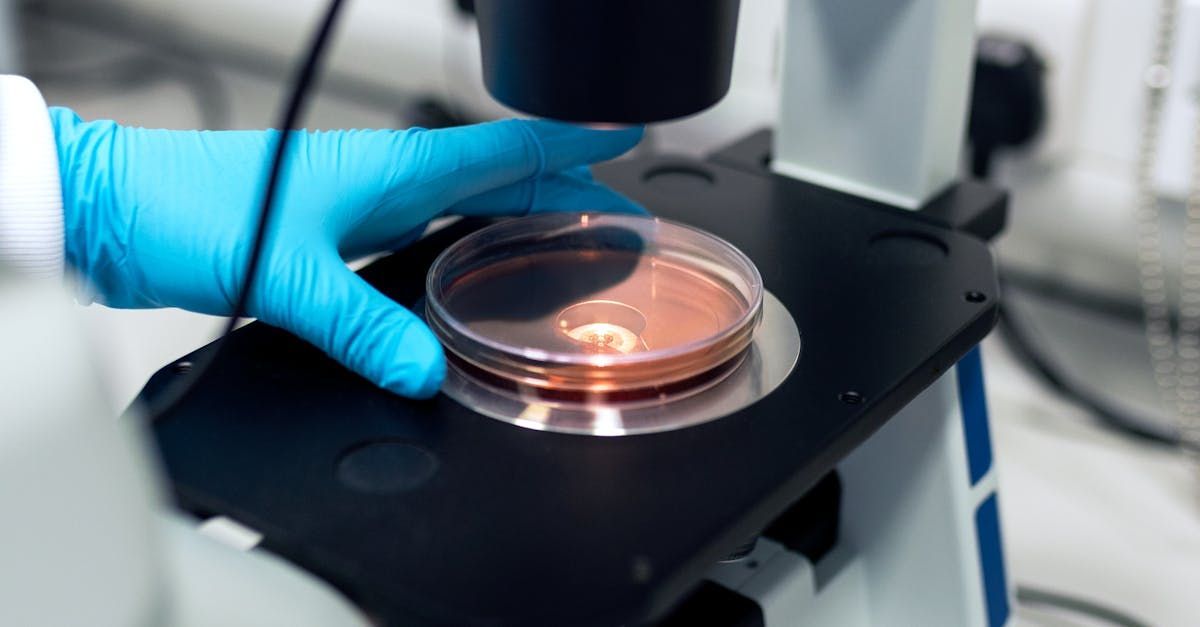
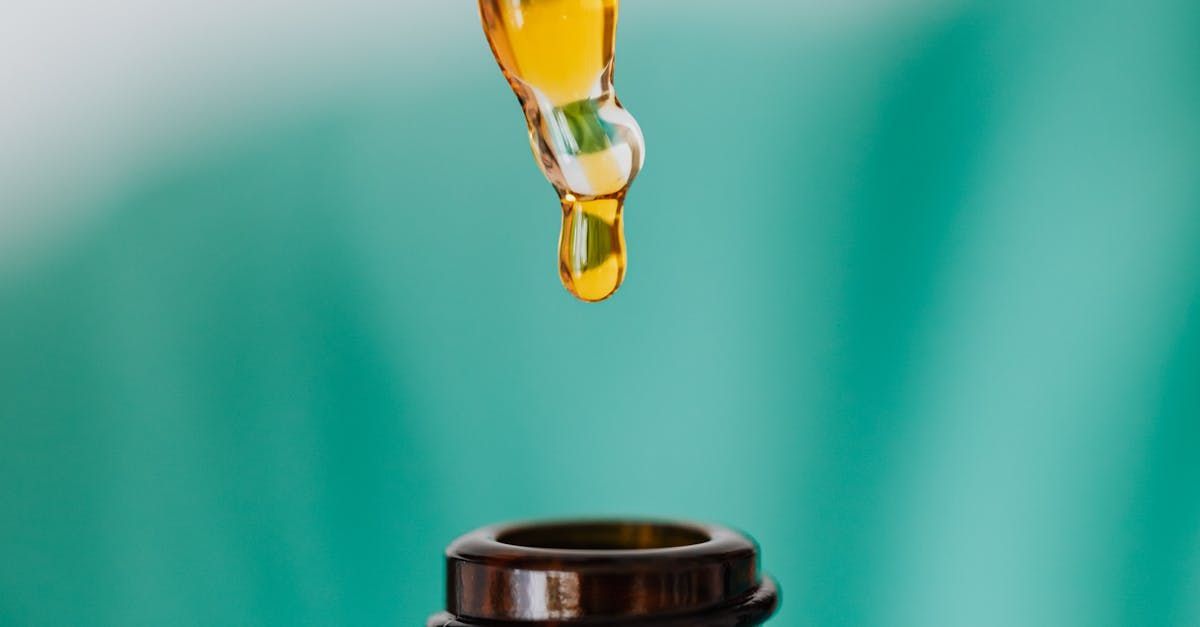
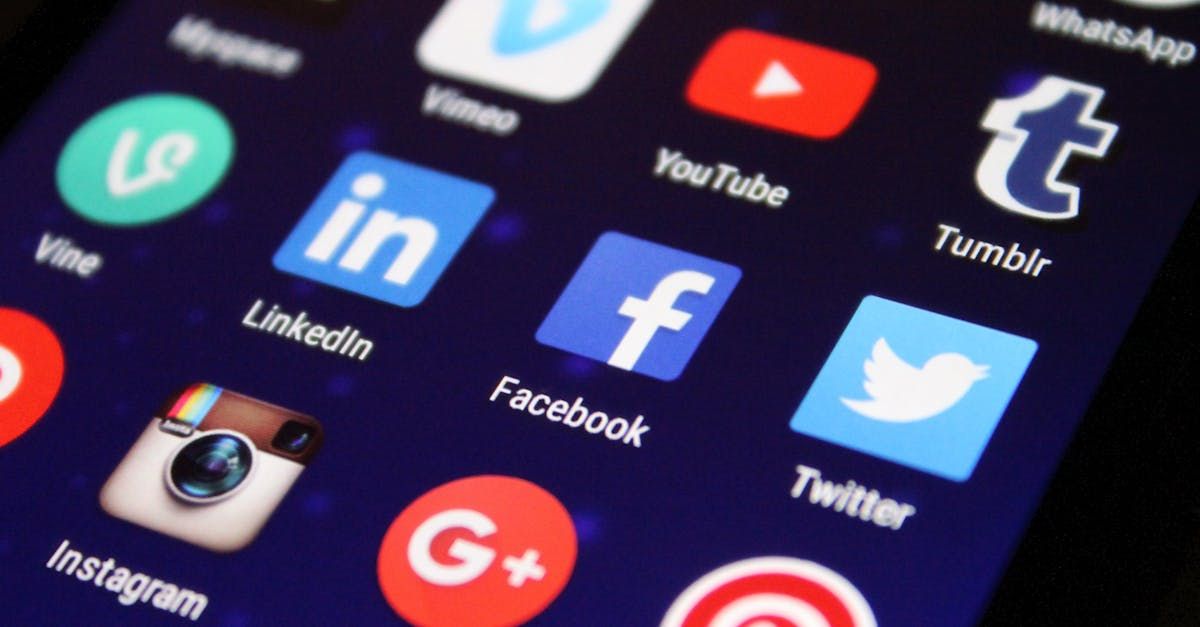